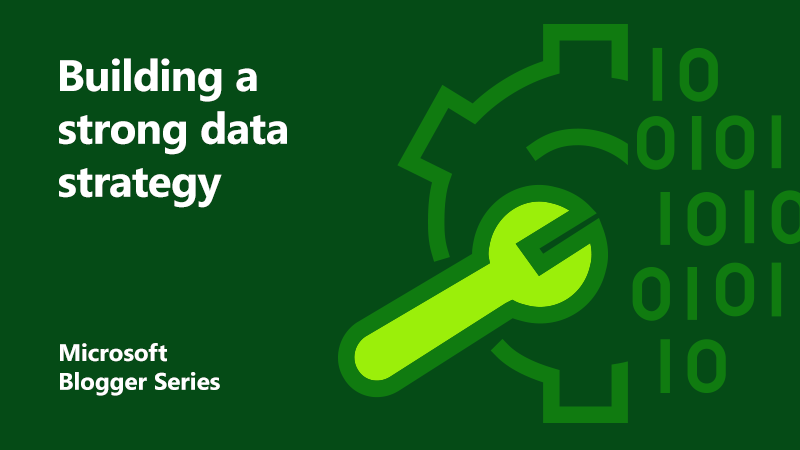
How to build and deliver an effective data strategy: part 1
Leveraging data to build better products and higher value services as a point of competitive advantage is nothing new. What is new is the volume, velocity, and variety of data that has been enabled by cloud computing. Designing a modern data analytics platform in the cloud is the convergence of security, governance, monitoring, on-demand scaling, data-ops, and self-service. What distinguishes a great data strategy from a good one is understanding how these facets interplay. We use tools like the Cloud Adoption Framework and the Well Architected Framework to ensure architectural cohesiveness, integrity, and best practices.
In this three part blog series, we’re going to guide you along the journey to build a strong data strategy. This first blog is designed as an introduction and will explain the core characteristics of data and some of the ways you can build competitive advantage through your data strategy.
Identify potential challenges and roadblocks to overcome
It can be difficult to harness the power of data in a secure and compliant manner. Sometimes, you can run into challenges like organisational silos, building a data-driven culture, and a never-ending challenge coming out of running multiple tools and technologies across the organisation. Time to market is one of the most critical factors these days for all businesses. Organisations can have great ideas and data can be an enabler, but with so many challenges it can take weeks, if not months, before they can start getting insights and ultimately deliver business value from data.
“An effective data strategy that delivers value to the business is based on four pillars: People with the right skills and data culture; Technology enabling speed of access to data; Processes to ensure security and repeatable patterns; Data the final golden ingredient that is trusted and understood. Get these right and the data strategy will fly.”
Peter Jackson, Director, Group Data Sciences at Legal & General, author of Chief Data Officers Playbook and Data Driven Business Transformation.
Understand the 7 attributes of data for a strong data strategy
To build a strong data strategy, you first need to understand how data works. Understanding these core characteristics will help you build a principled practice around how to deal with data.
1. Data travels fast, but the velocity of data movement cannot defy the laws of physics. It must conform to the laws of the land or the industry that created it.
2. Data never changes by itself, but it is prone to changes and accidental loss, unless explicit measures are in place to mitigate such challenges. Ensure controls, databases and storage anti-corruption measures, monitoring, audits, alerts and downstream processes are in place to deal planned or unforeseen changes.
3. Data by itself, and simply though the act of storing it, does not produce any insights or yield any value. In order to discover insights or extract value, most (if not all) data, independent of the volume, velocity, variety and veracity, has to go through four discrete steps: ingestion, storage, processing, and analytics. These each have their own set of principles, processes, tools, and technologies. Withholding data assets and related insights may affect socio-economic, political, research and investment decisions, hence it is of paramount importance for organisations to build the capability to provide insights in a secure and responsible manner.
4. All data generated or procured must go through a data classification exercise, unless otherwise explicitly stated. Where needed, the gold standard for dealing with confidential data is encryption at rest and in transit.
5. Data has gravity. This means that data, applications, and services all have their own gravitational pull. But data is the heaviest here, and therefore has the most gravitational pull. Unlike Newton’s apple, data doesn’t have a physical mass to draw in surrounding objects; instead, latency and throughput act as accelerants to the analytics process.
6. Latency, throughput, and ease of access often warrants that data is duplicated even when that is not the desired outcome. Set up people, processes, tools, and technologies appropriately to balance such requirements against organisation’s data polices.
7. The speed at which data can be processed is governed by architectural constructs, and facilitated through innovations in software, hardware, and networking. Some of the key architectural considerations are: setting up data distribution, partitioning, cache technologies, batch vs stream-processing, and balancing backend vs client-side processing.
Prioritise the business outcomes you want your data strategy to achieve
It is key to align your data strategy with your business outcomes. Having a successful data strategy will give you competitive advantage. In essence, most business outcomes can be classified under one or more of these four umbrella categories:
1. Empower your employees
Consider enabling your workforce with real-time knowledge of customers/devices/machines, efficiently collaborating to meet customer or business needs with agility.
2. Engage with customers
Deliver a personalised, rich, connected experience, inspired through your brand. Drive loyalty along every step of the customer journey by harnessing the power of data and insights.
3. Optimise operations
Increase the flow of information across your entire business operation. Keep your business processes synchronised and make every interaction valuable through a data driven approach.
4. Transform your products and development lifecycle
Gather telemetry data about your services and offerings. Use the data to prioritise a release or create a new feature, and evaluate effectiveness and adoption continuously.
Once you have prioritised your business outcomes, it is key to look at current projects, long-term strategic initiatives and classify them accordingly. Consider combining the 4 business outcomes in a matrix format shown below, based on complexity and impact. Also, think about adding the architectural pillars to help you dive deeper into the scenario.
Benchmark your current data estate and capability
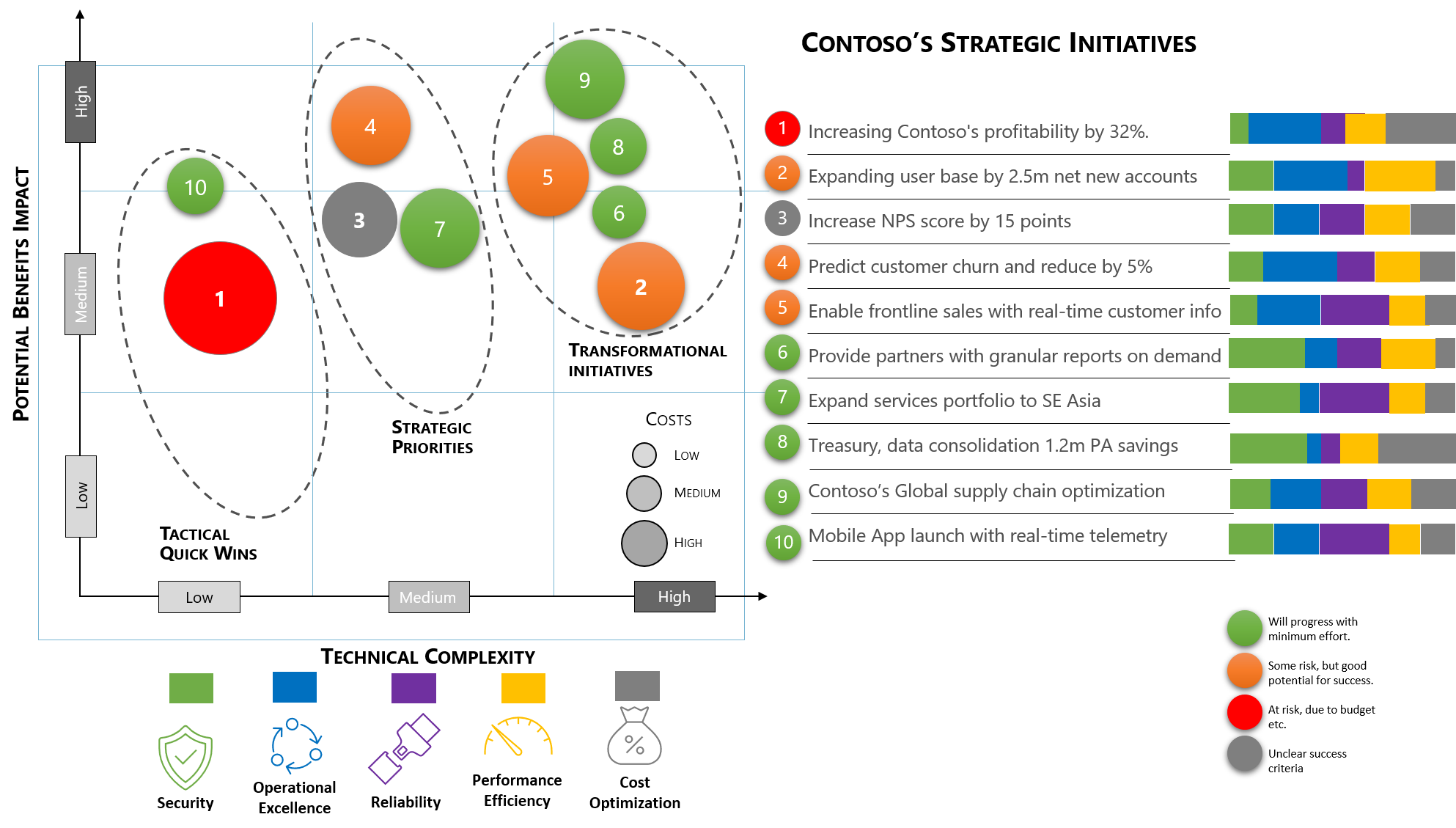
Figure 1: Aligning strategic business priorities with technical complexities
Benchmarking your current estate is critical to measure success. This allows you to quantify the exact investment needed in terms of people, process and technology. You’ll be able to see where you are on the Maturity Model, and the gaps you need to bridge.
Our teams work regularly with many customers on these and can help you get started. Here is a table showing the thought process:
Category | Action areas | Current Status | Focus on 5 pillars of Architecture |
Preparation | There are about 8-10 topics here starting from Strategy, Charter, Ethics, etc. | ![]() |
|
Agility | Depending on the organisation there could be 5 to 10 topics here, such as strategy around Data Lake, Catalogue, Common Data Model etc. | ![]() |
|
Resilience | 10-20 topics are here starting from discovery, recovery and anomaly detection. | ![]() |
Figure 2: Assessing your current estate and capability is key in ensuring you can track progress and celebrate success. Using the maturity level detailed in part 2 of this blog, you can rate your current capability 0-4 and use the 5 pillars of architecture to guide those benchmarks.
Once you’re clear about the business outcomes you’re looking to drive through your data strategy, the next step is to build the capability to deliver them. The second blog in this three part series takes you through how to build your data strategy with practical steps to map your capabilities, deliver a data-driven culture, and evaluate products and services. In part three, we dive deeper into how to execute on your data strategy.
[msce_cta layout=”image_center” align=”center” linktype=”blue” imageurl=”https://www.microsoft.com/en-us/industry/blog/wp-content/uploads/sites/22/2020/09/PreviewImage-2.png” linkurl=”https://www.microsoft.com/en-gb/industry/blog/?p=40388&preview=true” linkscreenreadertext=”How to build and deliver an effective data strategy: part 2 ” linktext=”How to build and deliver an effective data strategy: part 2 ” imageid=”40718″ ][/msce_cta]
Contribution thankfully received from Nick Hurt (Senior Solutions Architect, Big Data Analytics), John Mallinder (Principal Enterprise Data Architect), Blesson John (Senior Solutions Architect, Data & AI), Dave Lusty (Senior Solutions Architect, Data & AI), Susan Meldahl (Director Business Programs), and Sumi Venkitaraman (Senior Product Marketing Manager, Data & AI)
Find out more
Driving effective data governance for improved quality and analytics
Powering digital transformation at Microsoft with Modern Data Foundations
Designing a modern data catalog at Microsoft to enable business insights
Download: A Guide to Data Governance
Resources for your development team
Encourage your developers to get started with Microsoft data analytics
About the author
Pratim Das is the Director of Data & AI Architecture and CDO Advisory at Microsoft UK. Pratim and his team’s mission is to work alongside their customers in delivering insights and most importantly value from data, in achieving great business outcomes. Be it retail, financial services, manufacturing, health care or public sector, they have industry knowledge, and deep domain expertise to build a resilient data culture, and customer capability. Pratim’s special interests are around operational excellence for petabyte scale analytics, and design patterns covering “good data architecture” including governance, catalogue, privacy and data democratisation in a secure and compliant manner. Pratim brings over 20 years of experience both as a customer, and also working as a technology vendor building Data & AI services, with a key focus on building capability, products and solutions, that leads into fostering a data driven culture.