This content has been archived, and while it was correct at time of publication, it may no longer be accurate or reflect the current situation at Microsoft.
At Microsoft, our sales and marketing team receives millions of potential sales leads each year that they must follow-up with to determine how they can best meet each potential customer’s needs. Microsoft Digital has helped sales and marketing embed AI and machine learning into the lead-qualification process in our Dynamics 365 sales solution, quadrupling our sales effectiveness and creating an environment of optimized operations and empowered employees that helps drive our digital transformation.
At Microsoft, our sales and marketing team receives millions of potential sales leads each year that they must follow-up with to determine how they can best meet each potential customer’s needs. Microsoft Digital has helped Microsoft sales and marketing infuse AI and machine learning into the lead-qualification process, creating a more intelligent and efficient method for identifying customers who might purchase our products. The new solution, using AI and machine learning integrated with our Microsoft Seller Experience (MSX) which runs on Dynamics 365, allows our sales and marketing team to more efficiently address customers’ needs. It has also quadrupled our sales effectiveness and created an environment of optimized operations and empowered employees that helps drive our digital transformation.
Using AI and machine learning for digital transformation
AI and machine learning are quickly becoming important foundations for digital transformation in many organizations, including Microsoft. With recent data science advances and more easy-to-implement AI services, combined with the inundation of smart technology into almost every aspect of business, the terms AI and machine learning have entered the vocabulary of the business world and the general public.
Machine learning and AI are being used increasingly and are becoming an integral part of companies’ digital-transformation strategy. At Microsoft, we’re using AI and machine learning across our entire organization to digitally transform the way we do business.
We used these technologies for lead qualification to support two of the four outcomes of our digital transformation strategy at Microsoft:
- Empower employees. Supply our sales team with the tools that empower them to achieve their best.
- Optimize operations. Improve the lead-qualification process, making it more efficient and cost productive.
Our primary motivation was to create a more productive experience for our salespeople and a more focused sales and marketing follow-up process for our customers.
Examining sales and marketing at Microsoft
To generate global demand for our products and services, our marketing and sales organization collects leads when people request information or access tracked content with an online form. Our system collects leads by using marketing vehicles such as:
- Trial products, services, and subscriptions for products such as Microsoft Azure and Microsoft Office.
- Events such as conferences, webinars, and training sessions.
- Content downloads.
When someone signs up for a Microsoft product trial, sends us an email, or downloads content, the person or business becomes a lead for the purposes of sales and marketing. We market to between 5 million and 10 million leads per year. These names—which can be a company or a person—become records in our marketing and sales system. Our salespeople take the leads they receive from our marketing and sales system and work to convert the unknown prospective customer into a sales-qualified opportunity (SQO): a customer who is ready to buy Microsoft products or services.
As a sales organization, our primary goal is to be as effective as possible in taking unknown leads and converting them to Microsoft customers—users and purchasers of our products. We begin the process by identifying who the unknown entity is, by obtaining the name, organization, and other basic information. We then take this prospective customer and try to ascertain how likely they are to purchase Microsoft software. We do this by assessing qualifying information in a continuing process that attempts to move them from a prospect, to a lead, to an identified sales opportunity, and finally, to a sales win and customer success.
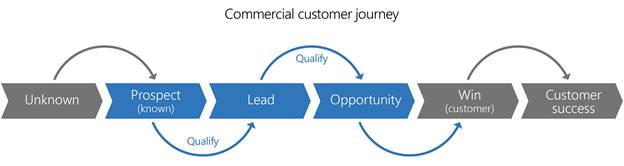
Historically, the leads that our salespeople receive come with very little qualification. Eighty percent of our leads do not respond to an initial contact from our salespeople. A lead might have been generated by using false or incomplete information, or a customer might have no intention of purchasing products when they download a product trial. As a result, our salespeople had to review up to 2 million leads annually to identify commercial customers who are ready to purchase a product. Leads that didn’t respond were abandoned, and salespeople pursued other leads on the list.
Our sales and marketing team knew that they needed a better way to qualify the massive amount of leads that our salespeople were processing. We wanted our salespeople to focus on selling. They were spending too much time trying to find prospective purchasers and not enough time focusing on customers who were ready to buy. We needed a more intelligent solution.
Initiating a more intelligent lead-qualification process
To improve the leads’ quality for our salespeople, we needed be able to define and measure that success. We established several goals that we wanted to achieve in improving the lead-qualification process by using AI and machine learning:
- Increase revenue. As a sales organization, our objective is to sell. If more customers buy our products and services, revenues increase, making Microsoft stronger and more profitable.
- Accelerate sales. Simply put, we wanted to move our customers from interest to product purchase more quickly. High quality, ready-to-buy leads remained untapped in our sales and marketing system because we had no way to move them to the top of the leads list.
- Increase sales system throughput. We wanted our salespeople to be able to process more leads. By processing up to 10 million leads per year, our salespeople worked through tens of thousands of leads each day. It took time to contact and dismiss leads that wouldn’t result in sales, and we wanted our salespeople to be able to recoup that wasted time and use it more efficiently.
- Improve the customer experience. We wanted to be able to better understand customers and ensure that their needs are met. This would require analyzing the information our leads contained more thoroughly and better understanding each lead’s underlying data.
Our primary measure of success for lead conversion is the rate at which our sales team converts a lead to a sales-qualified opportunity (SQO) in MSX: someone ready to purchase. Before we started using AI to qualify leads, our sales team was converting approximately 4 percent of the between 1 million and 2 million commercial leads annually into SQOs. We wanted to improve that rate significantly.
Considering AI for lead-qualification improvement
The journey into AI-driven lead qualification started before AI became part of our lead-assessment process, and it has occurred in three distinct phases:
- Business rules. This where we started, before AI was incorporated into lead qualification. Our sales and marketing system contained business rules that provided a relative value indicating the likelihood of a lead being converted to a sales opportunity. These rules assigned a point value to a customer to create a lead-scoring structure.
- AI-assisted lead scoring. During this phase, we implemented AI to improve our lead-scoring system. AI lead scoring focuses on customer behavior and assigns values to the different ways that a customer interacts with Microsoft, creating a more accurate scoring result.
- Bot-driven engagement and conversational intent detection. The most recent phase involved including an AI bot and a machine-learning-driven engine that examines and manages email communications between the customer and our sales team. We’ve named the engine Bot (and AI) Enabled Augmented Marketing (BEAM). BEAM focuses on using conversational intent detection to determine the likelihood that a customer is ready to purchase our products or services.
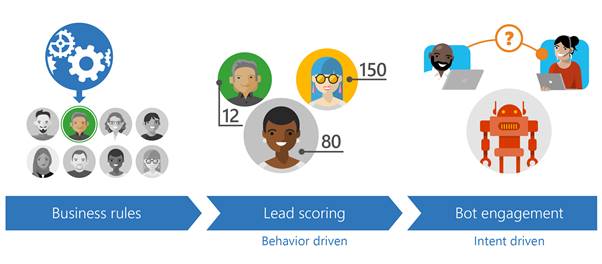
Examining our pre-existing basic business rules
Prior to AI and machine learning, we used basic business rules that we configured in our sales and marketing system to identify incoming leads. It provided a basic scoring system to help our salespeople choose leads that might yield sales opportunities. However, the scoring system’s business rules couldn’t account for the wide variety of lead quality and intent, so it still produced many leads that weren’t interested in purchasing Microsoft products. Our conversion rate to SQO under this system was approximately 4 percent.
Improving basic lead qualification with AI lead scoring
Our first step in improving lead quality was to implement AI-assisted lead scoring. We designed the scoring system to examine the different aspects of incoming leads and assign relative values to those aspects as they related to lead quality. AI lead scoring improved on the static, business-rules-based system by focusing on behavior and attributing a dynamic value to a lead based on that behavior. Our algorithm accounted for important information, such as:
- Demographic information, including region or industry.
- The lead’s role in their organization as an influencer or business decision maker.
- Prior history with Microsoft, including purchase history and contribution to product reviews and product feedback.
- Recent activity that the lead has performed, such as downloading additional content, responding to a Microsoft promotional email, attending a Microsoft event, or filling out an online form.
The system generated a score for each lead based on algorithm output, and then stored that information in our sales and marketing system. Leads given a higher score were pushed to the top of the list while lower scoring leads were placed at the bottom. The results provided our salespeople with a ranked list from which they could take the highest-scoring leads and engage the customer.
We designed the scoring to go deeper into business processes to create context around a lead, thus enabling our salespeople to better respond to the lead. It helped us begin to move from high-volume, low-quality leads to high-quality, scored leads. Our sellers could now focus on the leads that were most likely to result in an SQO. However, we recognized even more potential in AI to improve the lead qualification further. The scoring process often missed leads that didn’t fit into the typical lead-qualification categories, even though the customer was ready to buy. In the past, such leads remained at the bottom of the list, where a long time might elapse before a salesperson pursued them. It also left leads in the system that exhibited the behaviors of a potential customer but one who was not interested in purchasing. For example, someone studying for certification in one of our products might sign up for trial software and attend workshops but does not intend to make any purchases. While AI lead scoring improved our SQO efficiency from 4 percent to 6 percent, we recognized the potential to add more intelligence into our qualification process.
Facilitating intelligent intent detection using BEAM
BEAM is the centerpiece of our current AI-based lead-qualification solution. We built BEAM as an orchestration engine to capture incoming lead contact information through email and automate the contact process, determine lead intent by using machine learning, and provide a clearer lead-ranking process.
The basic BEAM process consists of the following steps:
- Take leads from our marketing and sales system and send an automated email asking how the salesperson might best help the customer.
- Ingest email response and process email data for intent detection. This involves preparation to ensure that the data is accurate and useable.
- Use machine learning to analyze email content and detect intent and context.
- Assign intent to a lead.
- Forward intent to a validation user interface to confirm intent classification.
- Process leads in preparation for injection into the sales and marketing system.
- Place the leads back into the sales and marketing system with appropriate intent and scoring information attached.
Understanding intent and context detection
BEAM’s intent-detection engine examines and identifies specific phrases as indicators of a customer’s intent to purchase products and services. For example, BEAM might recognize the phrase “wondering about pricing” as an indicator that a lead is ready to purchase. On the other hand, a phrase such as “Never contact me again” is a pretty clear indicator that a customer is not interesting in purchasing. BEAM uses machine-learning models to perform two assessments of the underlying data:
- Intent detection. Using natural language processing, intent detection assesses content and matches it with an intended task or direction. In the case of BEAM, we have four intent classifications for leads:
- Hot. A lead is classified as hot if the customer is ready to purchase. Usually language in the email, such as questions about pricing, licensing or availability, indicates clear intent to purchase. Leads classified as hot automatically go to the top of the sales queue.
- Not Interested. BEAM classifies a lead as not interested if there is clear indication that a customer is not ready to buy at this time but should remain in the system for potential purchase in the future. These leads are removed from the sales pipeline at the current time.
- Do not contact. If an email contains language indicating a clear desire for no contact or phrases that indicate anger or frustration, BEAM removes the lead from the sales pipeline and our marketing consent list.
- None of the above. If the lead doesn’t fall into clear intent categories, the lead is classified as none of the above and is taken out of the sales pipeline and, in most cases, the original message is redirected to an appropriate channel based on context.
- Context detection. We use context detection to provide another layer to BEAM’s decision-making capabilities. Many of the emails that come in through the BEAM bot are not from customers seeking sales help. They could be support requests, questions about feature education opportunities, solicitations, or other messages unrelated to the sales pipeline. Context detection uses natural language processing to determine what contexts are contained in a message and use those contexts for redirection, more detailed classification, or other decision-making capabilities.
Examining BEAM architecture and infrastructure
The BEAM platform is built on Microsoft Azure technologies and open-source AI and machine learning tools, including Microsoft Cognitive Services, Azure Machine Learning Server, and Azure Machine Learning Studio and then integrated into Dynamics 365. We built BEAM to be modular and flexible; we can interchange components and modify component behavior without negatively affecting the solution as a whole or refactoring.
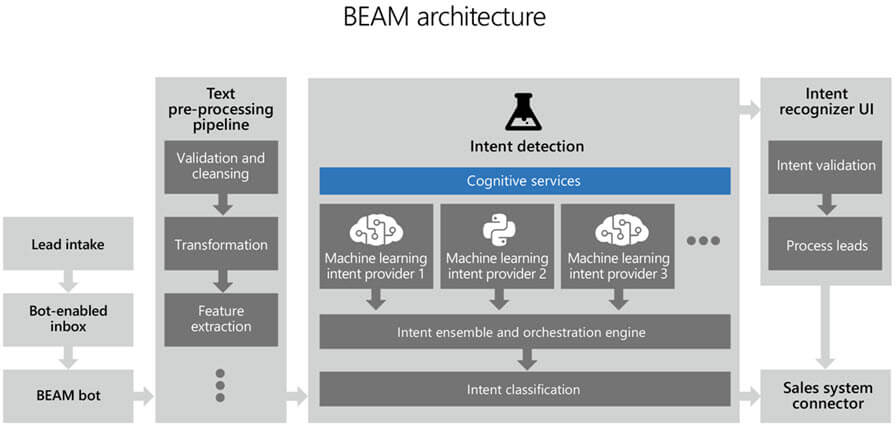
Ingesting and preparing data for machine learning input
One critical component of our BEAM architecture model is data preparation and cleanup. The machine-learning models for BEAM accept only plain-text inputs. The plain text is parsed in its entirety for intent and context detection. All BEAM inputs come from an email source, which has its own inherent data-quality issues when converted to plain text. Several parts of the email message must be stripped from the plain text input because they provide no value in intent detection and might cause issues with model-training behavior. These include:
- Email greetings, signatures, and sign-offs.
- Text included as part of a reply.
- New line characters, formatting characters, and other unnecessary characters.
We use Bing Spell Check in Microsoft Cognitive Services to spell check incoming email text to ensure proper recognition by the machine-learning models. We also created code to remove private personal information from ingested text.
Machine-learning architecture and models
At BEAM’s core are its machine-learning models. We use several models that each provide intent detection and work together to provide BEAM functionality.
Language understanding with LUIS
Our first model, and the one that does the most intensive work, is the Language Understanding Intelligent Service (LUIS) machine-learning service from Microsoft Cognitive Services. LUIS is a natural-language processing model designed to identify valuable information in conversations, interpret intent, and distill information from sentences for a high-quality, nuanced language model. LUIS integrates seamlessly with several API interfaces, which makes it extremely easy to incorporate into BEAM.
We created a layer in BEAM that sends API calls to LUIS by using JavaScript Object Notification (JSON). The JSON code is the primary input for LUIS, and it contains the prepared, cleaned data that LUIS ingests. A second layer translates the LUIS output into a standard response, in JSON, that is consumed by the rest of the BEAM architecture.
We’ve used LUIS to create applications in BEAM for both intent detection and context detection. LUIS’ out-of-the-box usability made it easy to quickly integrate LUIS-based applications into BEAM. LUIS also provides a flexible base for building machine-learning applications. Even though context detection isn’t one of the natively supported uses for LUIS, we were able to leverage the service to create an easy-to-integrate context-detection service for BEAM that could run alongside the intent detection engines (including LUIS) that we were using in BEAM. We’re continually examining LUIS for more opportunities to build other functionality into BEAM.
Custom-coded models from scikit-learn
We also implemented three separate models from scikit-learn, an open-source data mining and analysis tool set that performs machine learning in Python. We host each model in Azure Machine Learning Server, which enables us to deploy the model to whatever scale we require and keep our BEAM environment hosted entirely on the Azure platform. We’re using the following scikit-learn models:
- Multinomial NaÏve Bayes (MultinomialNB)
- C-Support Vector Classification (SVM.SVC)
- Linear classifiers with stochastic gradient descent (SGD) training (SGDClassifier)
Each of these models provides an evaluation of the input data from a distinct perspective and provides an equally weighted output that contributes to the determined intent.
Combining machine learning output with ensemble methods
Each machine-learning model produces a discrete result, indicating the evaluated intent from a lead email. We use ensemble methods meta-algorithms to assess all four models’ results and provide output to the BEAM engine from the model that generates the most accurate results. Ensemble methods allow us to freely insert and remove machine-learning models into the BEAM pipeline. We can test model effectiveness more quickly and adapt our machine learning practices according to our business needs.
We use the Ensemble Majority_MaxOrder method, which considers all model inputs and chooses the intent that received the majority vote from all models. If there is a contention (two intents have an equal number of votes), the one with the highest probability score is chosen. If several have the same probability score, the order of the models determines which one is chosen. We are continually evaluating the models’ output and considering other potential ensemble methods as BEAM intent detection matures.
Training machine-learning models and providing classification feedback
Our models are continually trained through an automated process that uses the previous month’s data and tests model output against the current month’s data. We also provide model training through our manual intent review portal, where our validation team can review BEAM output for validity and accelerate the training process.
This manual training process was extremely important in the early stages of BEAM implementation, and continued use helped our machine-learning models make more accurate predictions very quickly. We also have an automated review process that takes obvious intent phrases such as “Do not contact me” and assigns the appropriate classification.
Surfacing AI within Dynamics 365
Once we have an intelligent lead qualification score for every lead, it is passed on to our MSX system, running on Dynamics 365. Using Dynamics 365 as our sellers’ main productivity tool and surfacing intelligent insights into that tool creates the best of both worlds for our sales force. Dynamics 365 and AI operate together to give sellers a view of their prioritized workload based on the highest potential customers in the opportunity pipeline.
Sellers now have the information they need to optimize their activities in their day, week and month. Sellers have a personalized view of prioritize opportunities. These are opportunities that are unique to them based on the product they focus on and their country and location. And they get to see the entire BEAM conversation with the customer so that can understand the full context of the customers’ needs before they place a call to that customer. This is just a start. We will continue to infuse AI into MSX, augmenting our sellers’ capabilities, so they can focus on the most important aspect of their job: taking care of their customers.
Implementing BEAM at Microsoft
We completed BEAM implementation over a six-month period at Microsoft. We provided proof-of-concept (POC) data and results, and then ran a two-month pilot project with 180 customers in two regions. The pilot allowed us to production test our architecture and end-to-end processes. We then rolled out BEAM to the rest of our sales organization over the ensuing three months. In the time that we’ve had to review its effectiveness, the combination of AI lead scoring and BEAM has more than quadrupled our SQO conversion rate, from 4 percent to 18 percent in MSX.
Benefits
BEAM and AI-based lead scoring have radically changed our salespeople’s ability to identify and pursue productive sales leads and deliver Microsoft products and services to more customers far more efficiently. We’ve experienced several specific benefits from BEAM and AI-based lead qualification, including:
- A better sales environment. We’ve increased our sales conversion rate from 4 percent to 18 percent, and that means a stronger sales organization across the board.
- Increased revenue. Our salespeople are providing product and service sales to more customers because of the improved lead-qualification efficiency. They’re pursuing high-value customers who are ready to purchase products and services.
- Accelerated sales. We’re moving our customers from product interest to product purchase more quickly. High-quality leads are identified quickly, and our salespeople can deliver products and services more rapidly.
- Increased sales-system throughput. Our salespeople process more leads. They don’t waste time on low-quality leads or chase customers who aren’t interested in purchasing our products.
- Improved customer experience. Our customers get their needs met better and more quickly. We also have a better understanding of who are customers are and what they want.
Best Practices
- Clean your inputs. Machine learning thrives on clean, accurate data. We worked hard to ensure that our inputs to BEAM were as clean as possible before relying on BEAM output for lead qualification. We factor in legal compliance considerations such as General Data Protection Regulation (GDPR) and ensure that our customers’ information remains private and protected.
- Use engineering best practices. Modern machine-learning interfaces and design patterns are built on modern development practices such as continuous integration and continuous deployment (CI/CD), agile development, and effective testing and logging practices. Adopting these in BEAM made it easier to change and grow the solution with our business needs.
- Adopt a modular approach to machine-learning components. Machine learning is an ever-changing field, and new models and algorithms appear often. We designed BEAM as an open platform to allow the use of any machine-learning models in the lead-qualification pipeline. This allows for simple insertion, testing, and removal models and the potential expansion of BEAM functionality in the future.
Looking forward
We’re excited about future possibilities for BEAM and other AI and machine-learning-based development at Microsoft. We plan to improve and expand in several areas:
- Improved context detection. We want to dig deeper into our data and move from simple determinative results to deeper composite results. For example, we want to be able to detect not only that a customer is out-of-office, but also when the customer will return.
- Increased automation. We’re investigating ways to create additional automation levels within BEAM, such as enabling automated meeting scheduling for our customers with our salespeople to discuss and finalize product sales.
- Expanded languages. We want to expand BEAM to process the native language of any of our customers.
Conclusion
Infusing AI and machine learning into Dynamic 365 for the lead-qualification process has greatly improved the effectiveness of our sales team, creating a more intelligent and efficient method for identifying potential customers. AI lead scoring and BEAM integrated into MSX have significantly improved the effectiveness of our sales pipeline by providing our sales team with higher quality leads that are ready to buy our products and grow their relationship with Microsoft. AI and machine learning have enabled our digital transformation, allowing us to empower our employees to achieve more and optimize our lead qualification process.