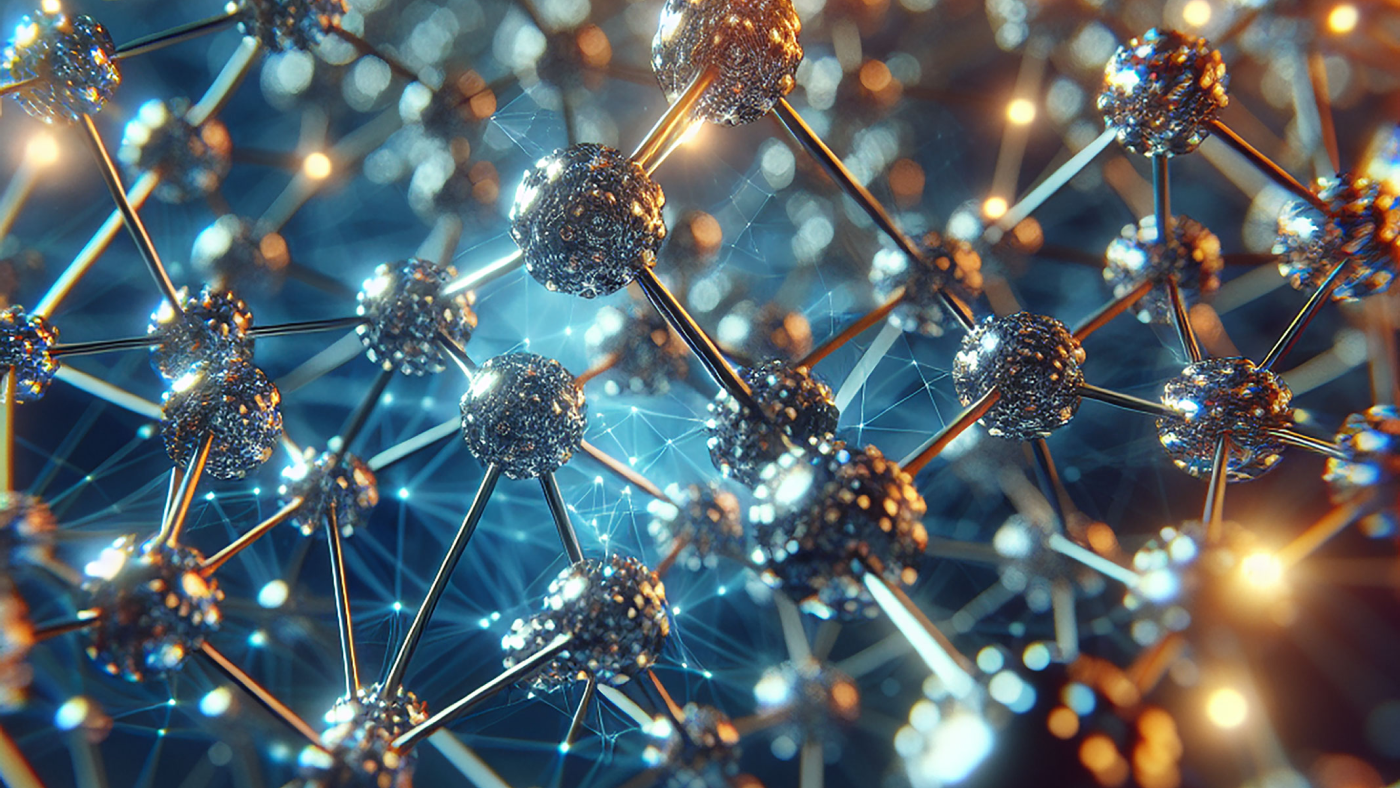
In the quest for groundbreaking materials crucial to nanoelectronics, energy storage, and healthcare, a critical challenge looms: predicting a material’s properties before it is even created. This is no small feat, with any combination of 118 elements in the periodic table, and the range of temperatures and pressures under which materials are synthesized and operated. These factors drastically affect atomic interactions within materials, making accurate property prediction and behavior simulation exceedingly demanding.
Here at Microsoft Research, we developed MatterSim, a deep-learning model for accurate and efficient materials simulation and property prediction over a broad range of elements, temperatures, and pressures to enable the in silico materials design. MatterSim employs deep learning to understand atomic interactions from the very fundamental principles of quantum mechanics, across a comprehensive spectrum of elements and conditions—from 0 to 5,000 Kelvin (K), and from standard atmospheric pressure to 10,000,000 atmospheres. In our experiment, MatterSim efficiently handles simulations for a variety of materials, including metals, oxides, sulfides, halides, and their various states such as crystals, amorphous solids, and liquids. Additionally, it offers customization options for intricate prediction tasks by incorporating user-provided data.

Simulating materials under realistic conditions across the periodic table
MatterSim’s learning foundation is built on large-scale synthetic data, generated through a blend of active learning, generative models, and molecular dynamics simulations. This data generation strategy ensures extensive coverage of material space, enabling the model to predict energies, atomic forces, and stresses. It serves as a machine-learning force field with a level of accuracy compatible with first-principles predictions. Notably, MatterSim achieves a10-fold increase in accuracy for material property predictions at finite temperatures and pressures when compared to previous state-of-the-art models. Our research demonstrates its proficiency in simulating a vast array of material properties, including thermal, mechanical, and transport properties, and can even predict phase diagrams.

Adapting to complex design tasks
While trained on broad synthetic datasets, MatterSim is also adaptable for specific design requirements by incorporating additional data. The model utilizes active learning and fine-tuning to customize predictions with high data efficiency. For example, simulating water properties — a task seemingly straightforward but computationally intensive — is significantly optimized with MatterSim’s adaptive capability. The model requires only 3% of the data compared to traditional methods, to match experimental accuracy that would otherwise require 30 times more resources for a specialized model and exponentially more for first-principles methods.

Spotlight: AI-POWERED EXPERIENCE
Bridging the gap between atomistic models and real-world measurements
Translating material properties from atomic structures is a complex task, often too intricate for current methods based on statistics, such as molecular dynamics. MatterSim addresses this by mapping these relationships directly through machine learning. It incorporates custom adaptor modules that refine the model to predict material properties from structural data, eliminating the need for intricate simulations. Benchmarking against MatBench (opens in new tab), a renowned material property prediction benchmark set, MatterSim demonstrates significant accuracy improvement and outperforms all specialized property-specific models, showcasing its robust capability in direct material property prediction from domain-specific data.
Looking ahead
As MatterSim research advances, the emphasis is on experimental validation to reinforce its potential role in pivotal sectors, including the design of catalysts for sustainability, energy storage breakthroughs, and nanotechnology advancements. The planned integration of MatterSim with generative AI models and reinforcement learning heralds a new era in the systematic pursuit of novel materials. This synergy is expected to revolutionize the field, streamlining guided creation of materials tailored for diverse applications ranging from semiconductor technologies to biomedical engineering. Such progress promises to expedite material development and bolster sustainable industrial practices, thereby fostering technological advancements that will benefit society.