As the means of expressing thoughts and feelings too sublime and elusive to be conveyed in everyday language, poetry across all cultures occupies a most sophisticated and mysterious of realms, just beyond the outskirts of creativity. Along the language-based avenues of expression available to people, poetry represents a departure from casual speech; few possess the talent to produce it. Yet successful poetry is instantly recognized and appreciated by millions when it is encountered.
To achieve true poetry then requires a very special and little understood brand of creativity. As difficult as it is for people to write it, imagine the challenge of designing artificial intelligence that might mimic the rare ability to produce poetic language in response to experience and existence.
Spotlight: On-demand video
A team of talented researchers at Microsoft Research Asia set out to attempt just that. In a paper titled “Beyond Narrative Description: Generating Poetry from Images by Multi-Adversarial Training (opens in new tab)” to be presented at the Association for Computing Machinery’s Multimedia 2018 conference in Seoul, South Korea, October 22–26, researchers Bei Liu and Jianlong Fu (opens in new tab) of Microsoft Research Asia in Beijing, along with teammates Makoto P. Kato and Masatoshi Yoshikawa of Kyoto University, took an imaginative approach to the quest of generating poetic language in response to images for automatic poetry creation, opening new possibilities for augmenting human endeavor. The project involved multiple challenges, including discovering poetic clues from images, as well as generating poems that would satisfy both relevance to an image and — something difficult to define but not disputed to exist — poeticness, to use the term coined by the researchers.
Automatic text generation from images is a field of research that has generated a lot of interest in recent years in, for example, automated image captioning and intelligent description and automatic sentence generation for images. These applications are concerned more with accuracy and utility. But compared with image captioning and paragraphing, things get very challenging when the objective is to wax poetic. Why? Relatively speaking, there is a philosophical chasm between mere visual representations and the poetic concepts and emotions that can be inspired in people by images that could then potentially be used to generate better — more poetic — poems. One illuminating figure in the ACM Multimedia 2018 paper illustrates the difference between mere textual description and a poem for the same image:
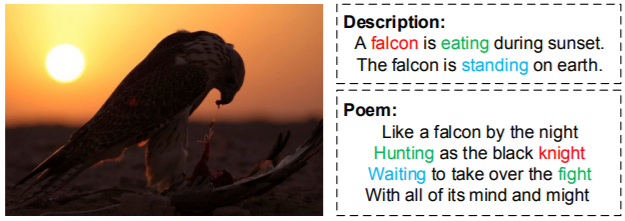
The difference between a human-written description and poem, based on the same image. Instead of describing the image factually, a poem tends to perceive a deeper meaning and poetic symbols from objects – knight from falcon, hunting and fight versus eating, and so on.
Understanding clearly what the challenge involved was central to the researchers’ success. “Generating a poem from an image is a cross-modality problem compared with poem generation from topics,” said Researcher Jianlong Fu. A tried-and-true and perhaps more intuitive way for poem generation from images is to first extract keywords or captions from the images and then use these as seeds for poem generation. However, as fellow researcher Bei Liu pointed out, keywords or captions don’t perceive a lot of the information in images — particularly the poetic clues that are important for poem generation. Compared with image captioning and image paragraphing, poem generation from images is an infinitely more subjective task.
The researchers also were aware that the style and form of lines in poetry are vastly different than those found in narrative sentences. The team made an early decision to concentrate on free verse, a much more open form of poetry, abandoning any requirements that the poem should rhyme or adhere to meter. This still allowed for a real sense of poetic structures and poetic language, what the team decided to call poeticness. Such poems by definition and the lines they contained wouldn’t be overly long. Word selections reflected words preferred by real poets versus the more mundane or literal words found in image descriptions. It also was acknowledged that specific words are preferred in poems compared with image descriptions and that lines in poems would maintain consistency to an overarching theme.
“The possibility of creating human-level content by AI requires building a deep and multi-modal understanding model spanning vision and language boundaries. Our team has consistently accelerated AI innovation to advance this dream.” – Jianlong Fu
Approaching their unique set of challenges, the researchers assembled two poem datasets using living annotators and decided on a methodology of poetry creation via integrating retrieval and generation techniques in one system. The team drove their methodology through extensive experimenting across over 8,000 images and evaluated the results using both algorithmic agents and people.
To better learn poetic clues from images for poem generation, they first learned a deep coupled visual-poetic embedding model with CNN features of images and skip-thought vector features of poems from a multi-modal poem dataset — MultiM-Poem — that consisted of thousands of image-poem pairs. This embedding model was then used to retrieve relevant and diverse poems from a larger uni-modal poem corpus — UniM-Poem — for images. Images with these retrieved poems and MultiM-Poem together constructed an enlarged image-poem pair dataset (MultiM-Poem (Ex)). The team took it a step further, deciding to leverage state-of-the-art sequential learning techniques for training an end-to-end image-to-poem model on the MultiM-Poem (Ex) dataset. This framework would ensure that the more robust poetic clues significant for poem generation would be discovered and modeled from the extended pairs. Two discriminative networks were used to provide rewards based on the generated poem’s relevance to the given image and its poeticness.
The generated poems were evaluated in both objective and subjective ways. The team defined evaluation metrics with regard to relevance, novelty and translative consistence and then conducted user studies to examine relevance, coherence, and imaginativeness of generated poems to compare its model to existing methods.
The point of this research is not to have AI replace poets. It’s about the myriad applications that can augment creative activity and achievement that the existence of even mildly creative AI could represent. Although the researchers acknowledge achieving truly creative AI is yet very far away, the boldness of their project and the encouraging results have been inspiring. To have set out to have a non-sentient machine take a run at a genre — English free verse — that is rather elusive even for motivated souls, whether you’re a besotted high school student or Bob Dylan himself, got the attention of fellow researchers in the space. That the early results aren’t on par with The Bard is beside the point; to the best of the researchers’ knowledge, theirs represents the first attempt to study the image-inspired English poem generation problem in a holistic framework, enabling a machine to approach people-level capability in cognition tasks.
They incorporated a deep-coupled visual-poetic embedding model and an RNN-based generator for joint learning in which two discriminators provide rewards for measuring cross-modality relevance and poeticness by multi-adversarial training. On the way, they built the first paired dataset of image and poem annotated by people, pairing it with the largest public poem corpus dataset. Extensive experimentation demonstrated the effectiveness of their approach, relying on objective and subjective evaluation metrics, including Turing testing it on over 500 people, including 30 poetry experts! In a spirit of sharing and to encourage further research in poetry generation from images, the team has released their datasets and code on Github2 (opens in new tab).
“It’s important to point out that we didn’t define what poeticness is, which actually is difficult to define,” said Liu. “We tried to make the machine learn both from poems and non-poems, so it could distinguish whether generated sentences were in a poem style or not.”
The results of the efforts are both fascinating and encouraging. Both subjective and objective evaluations showed superior achievements against existing state-of-the-art methods for poem generation from images.
“We have introduced a new artist. And I hope he/she can help prompt more people’s interest in art.” – Bei Liu
Generation of poems isn’t something that hasn’t been tried. XiaoIce, the Microsoft advanced natural language chatbot, has been dabbling in Chinese poetry aimed at entertaining Chinese users for a couple of years now. But XiaoIce relies on keywords to generate poetry, unlike the research model pursued by this team that generates poems directly from images using an end-to-end approach without relying on keywords as a midlevel result.
Again, why the quest to achieve machine-generated poetry? There are both aesthetic and commercial motivations. Fu sees applications of any advances in creative abilities in AI as augmenting human creativity in spaces such as gaming, image generation, and the fashion industry. “To be honest, the current creative capability of AI is still far from that of people,” said Fu. “But we believe AI could be a type of assistant ‘who’ can help to reduce redundant work in design for artists and designers in the future.”
Parallel research
What are the researchers looking at next? Storytelling. Their current project is looking to generate a story from multiple images — they call it visual storytelling. Right now, things are limited to the generation of general sentences, limited to a dataset. Fu and Liu are trying to introduce extra signals into the mix, such as emotion, to generate the stories. Building a model to simulate the human emotions and given a picture, they will first generate a distribution of emotions; indeed, there can be multiple related emotions to an image because every individual may have different views based on experience, culture, and identity. The model would automatically sample a specific emotion from the distribution and then use this signal as an input to generate the final story. “This could enhance the model to generate more diverse stories and thus resemble something more created by an individual,” said Fu. “We are trying to incorporate random signals in training the model to create a more unique machine, that is, one more resembling a subjective individual.”
Liu pointed out that the machine’s eventual individuality may be unavoidable. “Although we currently are training the machine to simulate how most people might respond, the machine nevertheless progressively learns from its own subjective experience, just as do individual people.”
Moved by AI
And was there a favorite poem for Liu, among all the AI created during the study?
Liu smiled. “It was this one,” she said, pasting a poem along with the image that inspired it (see below.)
The sun is shining
The wind moves
Naked trees
You dance
“This is a poem simple in language, inspired by a very common image we might easily glimpse in daily life,” she said. “And yet it seems real.” She draws our attention to the ambiguity of the word “you” in the final line. “It could be the tree. A friend. Even myself. I think this is the magic of poetry, and our work has the potential to create that magic.”