One empirical finding in deep learning is that simple methods such as stochastic gradient descent (SGD) have a remarkable ability to fit training data. From a capacity perspective, this may not be surprising— modern neural networks are heavily over-parameterized, with the number of parameters much larger than the number of training samples. In principle, there exist parameters to achieve 100% accuracy. Yet, from a theory perspective, why and how SGD finds global minima over the set of non-convex and non-smooth neural networks remains somewhat mysterious.
Our team of researchers at Microsoft Research in Redmond, Washington resolved to shed some light on this mystery. Our findings are available on arXiv.
Our main finding demonstrates that, for state-of-the-art network architectures such as fully-connected neural networks, convolutional networks (CNN), or residual networks (Resnet), assuming there are n training samples without duplication, as long as the number of parameters is polynomial in n, first-order methods such as SGD can find global optima of the training objective efficiently, that is, with running time only polynomially dependent on the total number of parameters of the network.
To better understand this finding, the following picture illustrates the main technical contribution of this our work. With the help of over-parameterization, surprisingly, there won’t be local minima blocking the SGD training; this means that as long as the training objective is large, its gradient—that is, backpropagation—also is large. However, this theorem alone does not give us the convergence result, because a neural network with ReLU activation is not even smooth. This suggests that myopically following the (opposite) gradient direction can, in principle, even increase the objective value. Fortunately, the next theorem in our paper showed that over-parameterization also makes the network “semi-smooth.” This implies if one moves in this opposite gradient direction, the objective value can indeed decrease. These two theorems together imply that SGD finds global minima on over-parameterized neural networks.
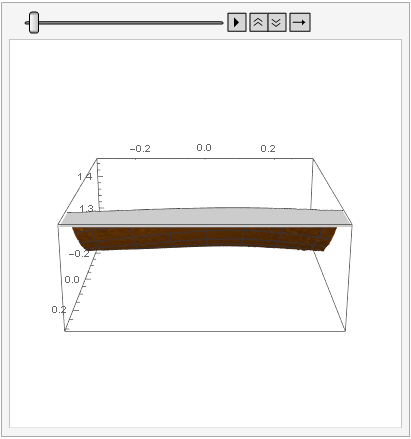
Microsoft Research Podcast
Collaborators: Renewable energy storage with Bichlien Nguyen and David Kwabi
Dr. Bichlien Nguyen and Dr. David Kwabi explore their work in flow batteries and how machine learning can help more effectively search the vast organic chemistry space to identify compounds with properties just right for storing waterpower and other renewables.
Landscape of neural network objective near the SGD training curve.
So, what else can we train using SGD, provably? This article only covers feedforward neural networks, such as CNN or ResNet. What about other more complex deep learning architectures? How about recurrent neural networks (RNNs), widely used in natural language processing? RNN is more difficult to analyze than DNN; indeed, we devoted a paper to addressing its training convergence.
Finally, what about getting good accuracy on testing data? This article only covers finding global optimization for the training data. How does the trained network generalize to test data? To properly answer this question, we teamed up with University of Wisconsin–Madison Professor Yingyu Liang to work out a generalization theory for such over-parameterized neural networks trained by SGD. The theory currently only works for neural networks with less than four layers, but more discoveries in deep learning are awaiting!
We would like to express thanks to Microsoft Research colleagues Harry Shum, Ofer Dekel, Sébastien Bubeck and Greg Yang for helpful conversations that made this work possible.