Academic research plays such an important role in advancing science, technology, culture, and society. This grant program helps ensure this community has access to the latest and leading AI models.
Brad Smith, Vice Chair and President
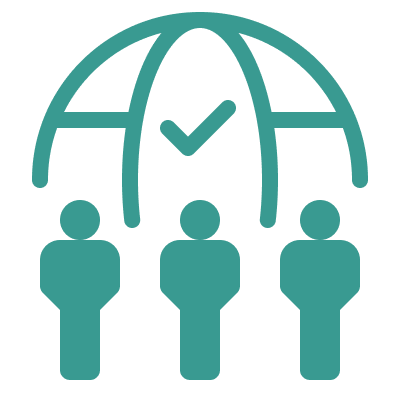
AFMR Goal: Improve human interactions via sociotechnical research
which increases trust, human ingenuity, creativity, and productivity, and decreases the digital divide while reducing the risks of developing AI which does not benefit individuals and society
These projects examine how AI can be used across different disciplines to support human creativity, improve workflow efficiency, and enrich the user experience. Some of the notable projects include using these models to inspire human creativity, and applying AI-transformed applications for immersive learning, coaching, and language learning. Moreover, proposals aim to improve the efficiency of AI-powered agents with advanced language communication tools and explore how AI can enhance creativity in virtual reality settings. Another interesting venture is to look into how AI can make academic insights more accessible for practitioners, and how it can comprehend and utilize emotional intelligence. Methodologies range from host studio sessions, design workshops, experiments and computational frameworks, among others. We expect these initiatives to lead to innovations that can address the current challenges, provide tools that can improve user experience and facilitate learning and creativity, and new discoveries in the understanding of AI’s emotional intelligence ability.
-
Cornell University: Jeffrey Rzeszotarski (PI)
This project outlines two experiments aimed at understanding how generative models can support human creativity. It explores the integration of DALL-E 2 into a creative drawing lab experiment and how GPT-4 can be integrated into an existing qualitative thematic coding tool to enhance user experience. By focusing on these successful AI models, it seeks to mitigate the potential risks of their application in creative tasks.
-
The University of Texas at Arlington: Cesar Torres (PI)
Bricolage is a creative practice emphasizing the influence of a practitioner’s environment (physical, social, or otherwise) on their creative process. In contrast to an empty room or screen, bricolage posits that spaces filled with possibilities (e.g., via galleries of artifacts, varieties of tools, diverse peoples) encourage improvisation, innovation, and resourcefulness; however, such spaces often evolve over decades of practice. Large language models offer a unique opportunity to generate conceptual resources to enhance bricolage practice across a variety of disciplines. Leveraging our current work on harvesting video tutorial transcripts from 30 communities of practice, this work aims to tune foundational models into practice-tuned LLMs (e.g., ElectronicsGPT, 3DPrintingGPT, CeramicsGPT, PromptGPT). These models will be used to extract and organize information about techniques, tools, and materials into accessible formats, such as dynamic AI-generated image collages and flow charts. This approach will offer a collection of bricolage resources to enhance human creativity, promote interdisciplinary innovation, and serve as a bridge for more practitioners to leverage AI within their respective practices.
-
IIT Tirupati: Sridhar Chimalakonda (PI)
Moving away from the predominantly common approach of hand-crafting source code representations [e.g. Abstract Syntax Tree (AST), Control Flow Graphs (CFG)] and AI pipelines for a given software engineering task (e.g. Code Summarization, Bug Localization, Code Clone Detection), the proposed project aims to find the appropriate mix of spruce code representation for a given SE task. Specifically, the proposed project builds on our mocktail approach and aims to create a a framework that can facilitate configuring and experimenting with different types and combinations of source code representations and ML models for various SE tasks. We see that this framework can help researchers and practitioners to explore, experiment and build an appropriate AI pipeline for a given SE task without manually creating each instance of the AI pipeline.
-
Prairie View A&M University: Malachi Crawford (PI)
Briefly, this research project seeks to evaluate the use of large language models, such as GPT-4 and DALL-E 2, in the creation of a stage play from primary source data. Through a process of AI prompting and iteration, we will develop a rubric to evaluate the model’s performance across five indices: character development, character dialogue, plot structure, set design/visual elements, and stage direction. Ultimately, we anticipate AI diminishing the barriers, such as time spent writing dialogue, scene creation, outlining stage direction, and other highly-skilled artistic activity that might constrain students of history from bridging the historical profession with the creative and performative arts. In so doing, this proposal aligns with the Advance Beneficial Applications of AI research component.
-
William & Mary: Yixuan Zhang (PI)
This proposal aims to investigate the capacity of LLMs to understand and harness emotional intelligence. The research expands emotional stimuli used in LLMs, assesses the depth and duration of emotional interaction with LLMs, and its influence on people’s perceptions and trust.
-
University of Missouri-Kansas City: Shu-Ching Chen (PI)
This research proposal aims to investigate the operational dynamics of Large Language Model (LLM) powered agents and their efficiency in task coordination. The primary focus areas of the study involve understanding the mechanisms that drive these agents, their specialized use of prompts and tools, knowledge sharing, and the role of a Knowledge Query Manipulation Language (KQML) inspired language in enhancing communication between these agents. The research aims to contribute additional knowledge to the field of LLM applications and explores the possibility of improving task efficiency and coordination. Moreover, the research intends to establish a foundation for future implementations in various applications requiring advanced task reasoning.
-
University of Illinois, Chicago: Nikita Soni (PI)
The long-term goal of this project is to design intuitive and natural collaborative child-AI visual storytelling interfaces for children’s creativity support. Researchers have made multiple efforts to enhance the efficiency of human-AI interactions in creativity support tools through algorithmic advancements. However, understanding how users of all ages, including children, interact with these creativity interfaces is limited. The output of this proposal will be a publicly available prototype and evidence-based design guidelines to inform the design of future AI-based visual storytelling interfaces for children.
-
Carnegie Mellon University: Sarah Fox (PI)
This proposal focuses on how queer artists leverage generative AI, highlighting the importance of considering queer people as users of GMs rather than solely targets of harm. The study will work with artists over multiple weeks to host studio hours and facilitate design workshops to understand challenges encountered by queer artists while using GMs and identify potential solutions.
-
Simon Fraser University: Steve DiPaola (PI)
The project aims to develop a next-generation AI conversational virtual human character and generative systems to enhance immersive training and coaching in various sectors. The research proposes to further AI visual agents and text-based conversational agents to facilitate language learning, job training simulations, health coaching, and real-time guidance.
-
University of Notre Dame: Diego Gomez-Zara (PI)
This proposal explores how LLMs can support human-AI collaboration in creativity tasks within virtual reality (VR) environments. It aims to comprehend the social and psychological effects of large language models on team creativity in virtual environments. The goal is to develop a computational framework to implement LLMs for VR environments, replicating the physical sequences for AI agents in human-AI teams.
-
University of Washington: Gary Hsieh (PI)
The proposal focuses on leveraging Generative AI to translate scientific insights from publications into more accessible forms for design practitioners, specifically through ‘design cards’. Using Microsoft Azure, they aim to further develop an initial version of a system that can convert academic papers into design cards, which has shown promise in preliminary studies. The research will investigate personalized translational resources, trust issues in AI-translated work, and real-world applications of AI-generated design implication cards.
Related paper: