AI for Precision Health
Explore how we leverage real-world observational data to optimize health delivery and accelerate biomedical discovery.

Our vision
At Microsoft, we aspire to advance AI toward developing a continuous learning health system (opens in new tab) that can instantly incorporate any new information to optimize delivery and accelerate discovery. In reality, the health system is mired in overwhelming unstructured data and non-scalable manual processing. Recent advances in generative AI such as large language models (LLMs) offer unprecedented “universal structuring” capabilities that can supercharge health information processing and unlock many high-value applications in real-world evidence and precision health.
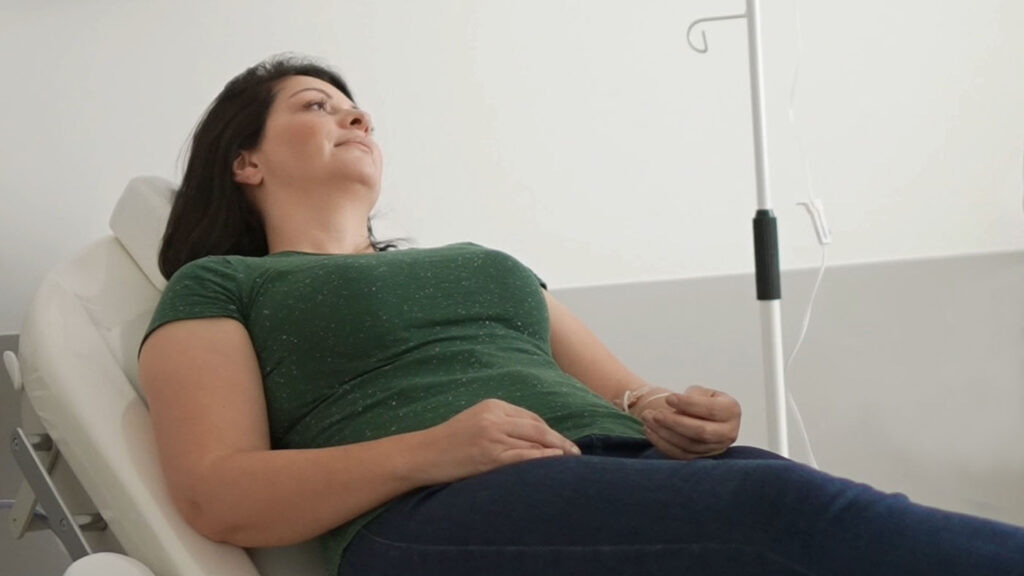
What does it mean for patients?
In joint work with large health systems, we have attained promising results in applying LLMs to structure clinical notes and other biomedical text at scale, with initial success in real-world applications such as molecular tumor board and clinical trial matching.

What does it mean for research & discovery?
Structuring longitudinal patient journeys at scale can drastically accelerate biomedical research and discovery. For example, by tallying millions of patients who have taken immunotherapies, we can compare exceptional responders against non-responders, and uncover novel opportunities in precision oncology.
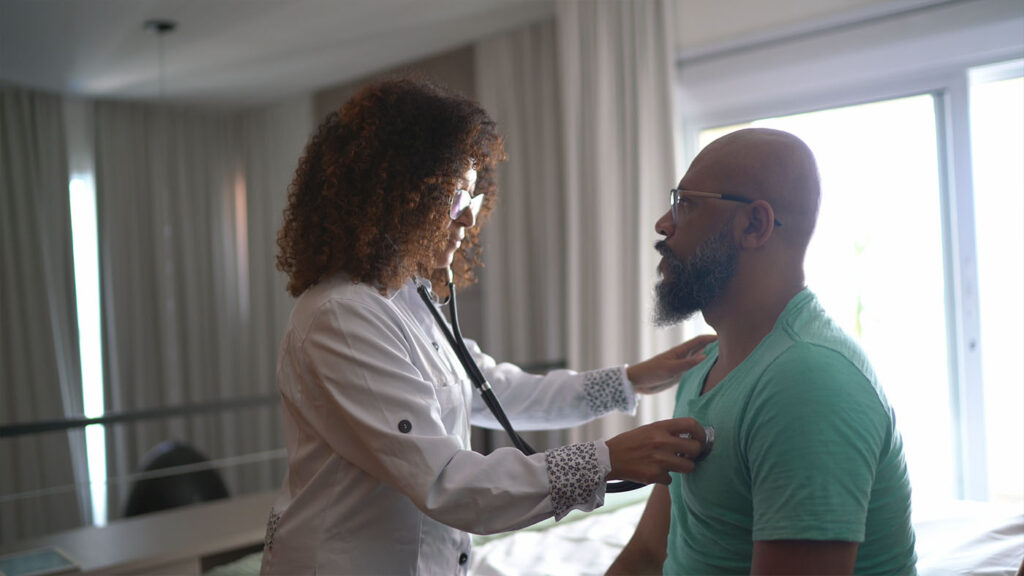
What does it mean for clinical practitioners?
By structuring the latest research findings and enabling patient-like-me search at scale, health LLMs can greatly empower clinical practitioners in pursuing evidence-based medicine. By accelerating case authoring from real-world use cases and offering learning copilot experience, LLMs can also help transform medical education.
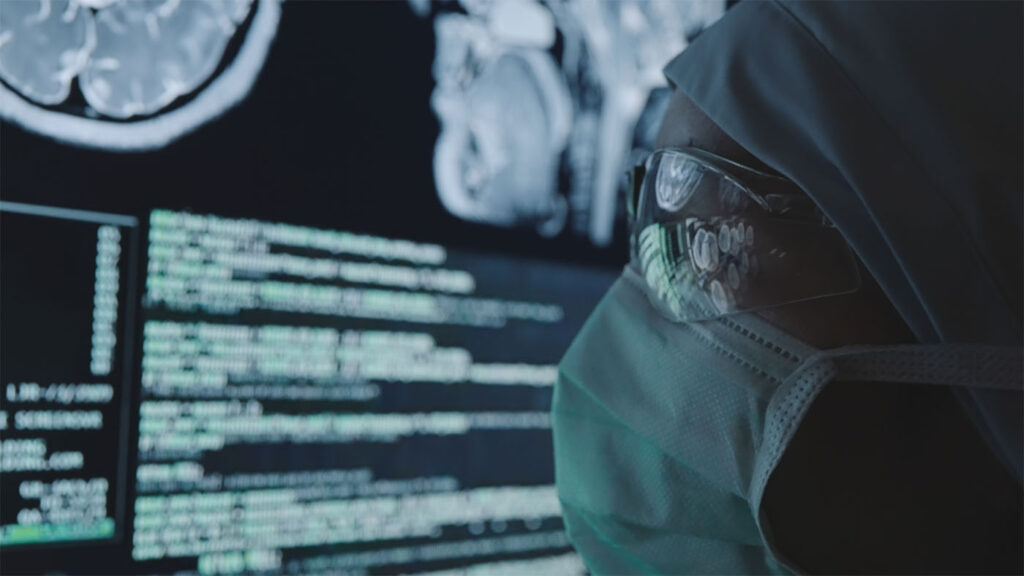
The future of precision health
Imagine the future when clinical research and care are seamlessly integrated. Every clinical decision is supported by patient-like-me information at the population level. Biomedical researchers can access world-wide real-world evidence in real time. Payors and regulators make approval and value-based care decisions based on comprehensive and up-to-date data.
Foundational research
The advent of powerful general LLMs heralds a new era of accelerated progress in precision health AI research and applications. Next-gen models such as GPT-4 provide strong out-of-box performance and can serve as a “universal labeler” for evaluation and supervision. However, while these models already have strong health competency from consuming publicly available biomedical text, there are salient growth areas in accuracy, safety, compliance, cost, explainability, to name a few.
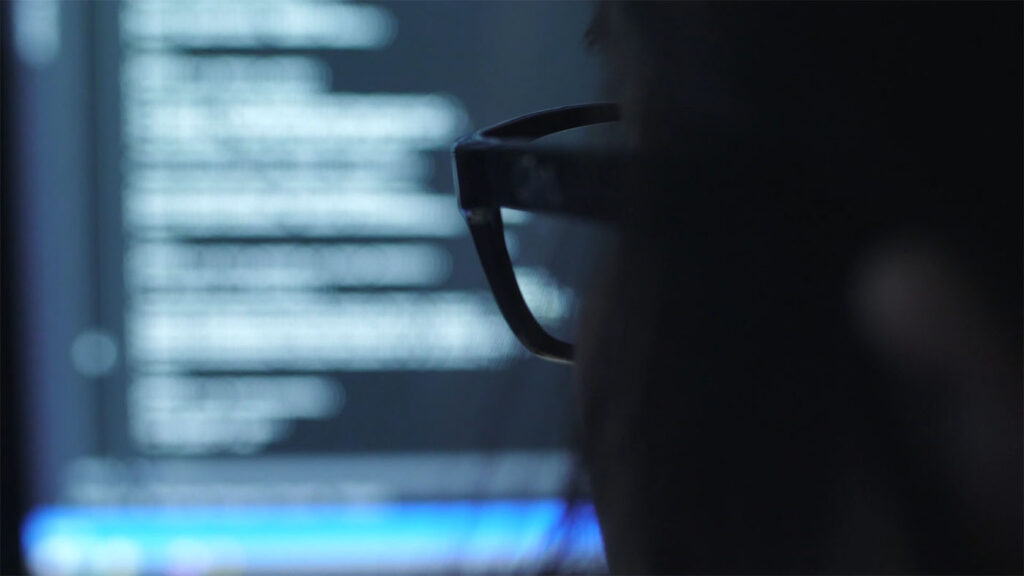
Prompt programming
LLMs usher in a paradigm shift toward prompt programming, which democratizes programming from programmers to general stakeholders. In health, a major bottleneck for adoption is accuracy. Our research improves safety guarantees via self-verification, by leveraging the insight that verification is often easier than generation (akin to P vs NP).

Model adaptation
LLMs such as GPT-4 can serve as a universal annotator to create large-scale synthetic data to distill domain-specific models and self-supervise multimodal instruction tuning. We are exploring such health-specific adaptations in structuring, summarization, QA, with significant advantages in cost, efficiency, and white-box model access.

Multimodal learning
For health applications, the biggest blind spot of web-based LLMs is multimodal, longitudinal patient data. We are exploring health-specific generative learning, with promising results using public data such as radiology (opens in new tab), single-cell (opens in new tab), biomedical literature (opens in new tab). A moonshot aspiration is multimodal fusion for predicting immunotherapy drug response.

Causal reasoning
An Achille’s heel of real-world evidence is confounders in observational data. By incorporating causal reasoning state of the art, we have developed a real-world evidence playground for scalable hypothesis generation and testing, such as simulating cancer trials using real-world data in silico.
From research to real-world impact
The health system is incredibly complex. Technologies such as generative AI, as powerful as they are, would only serve as the catalyst. Meaningful progress can only be attained by embedding research and development into end-to-end applications, in deep collaboration with all stakeholders, with accuracy, safety, and compliance being first-class citizens. We conduct our research in tight collaboration with key stakeholders from delivery to discovery and are excited to see promising progress along the way.
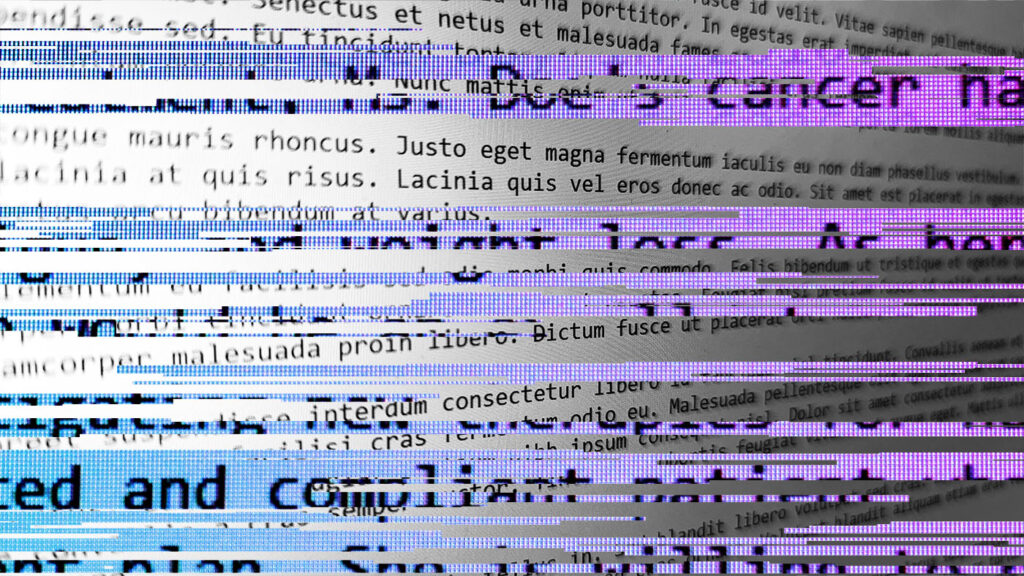
Unstructured data: “Dark Matter” in the RWE universe
Prior work in real-world evidence is largely restricted to available structured data, such as claim codes, which severely limits applicability. By accelerating clinical abstraction, we can unlock “dark matter” in the unstructured data and propel real-world evidence generation to the next level.
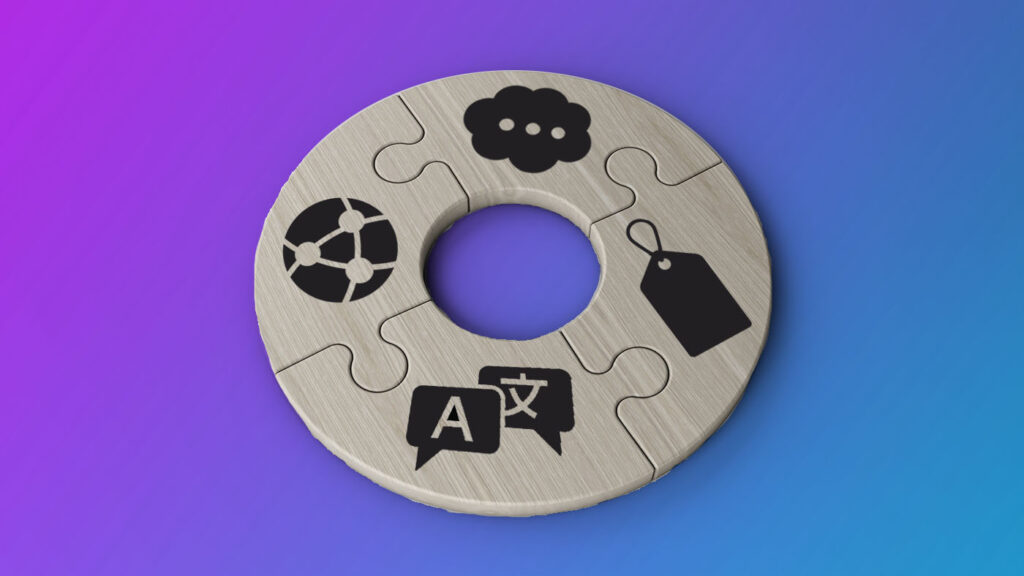
Precision health in the age of LLMs
The advent of LLMs sets new patterns in precision health: “universal structuring” scales real-world evidence generation; “universal labeling” scales evaluation and model adaptation; “universal translation” lessens burden in interoperability; “universal reasoning” provides interpretable rationale, facilitates human-in-the-loop verification, and scales discovery.
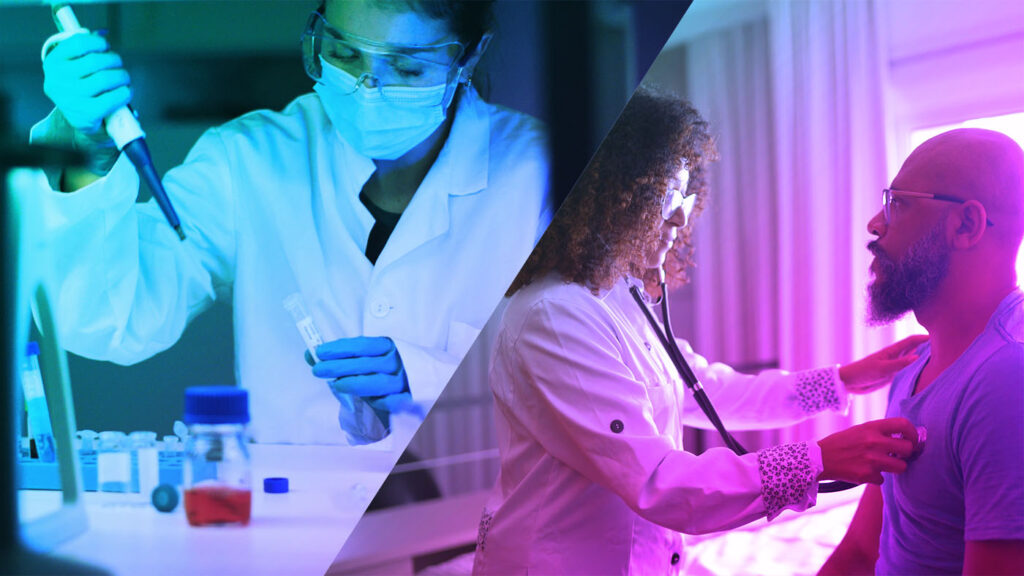
From discovery to delivery
Precision health applications can be loosely divided into care/delivery and research/discovery. While accuracy is important across the board, the bar is significantly higher in delivery. Our research prioritizes discovery initially, but the same technical advances are equally applicable to delivery.