In a few years, the world will be filled with billions of small, connected, intelligent devices. Many of these devices will be embedded in our homes, our cities, our vehicles, and our factories. Some of these devices will be carried in our pockets or worn on our bodies. The proliferation of small computing devices will disrupt every industrial sector and play a key role in the next evolution of personal computing.
Most of these devices will be small and mobile. Many of them will have limited memories (as small as 32 KB) and weak processors (as low as 20 MIPS). Almost all of them will use a variety of sensors to monitor their surroundings and interact with their users. Most importantly, many of these devices will rely on machine-learned models to interpret the signals from their sensors, to make accurate inferences and predictions about their environment, and, ultimately, to make intelligent decisions. Offloading this intelligence to the cloud is often impractical, due to latency, bandwidth, privacy, reliability, and connectivity issues. Therefore, we need to execute a significant portion of the intelligent pipeline on the edge devices themselves.
Modern state-of-the-art machine learning techniques are not a good fit for execution on small, resource-impoverished devices. Today’s machine learning algorithms are designed to run on powerful servers, which are often accelerated with special GPU and FPGA hardware. Therefore, our primary goal is to develop new machine learning algorithms that are tailored for embedded platforms. Rather than just optimizing predictive accuracy, our techniques attempt to balance accuracy with runtime resource consumption.
We are taking two complementary approaches to the problem. The first is a top-down approach: we design algorithms that take large and accurate existing models and attempt to compress them down to size. Specifically, we are focusing on compressing large deep neural network (DNN) models, with applications such as embedded computer vision and embedded audio recognition in mind, and exploring new techniques for DNN compression, pruning, lazy and incremental evaluation, and quantization. The second approach is from the bottom up: we start from new math on the whiteboard and create new predictor classes that are specially designed for resource-constrained environments and pack more predictive capacity in a smaller computational footprint.
Edge intelligence as found in embedded devices is typically supplemented with additional intelligence in the cloud. Therefore, we are developing algorithms that dynamically decide when to invoke the intelligence in the cloud and how to arbitrate between predictions and inferences made in the cloud and those made on the device. We are also developing techniques for online adaptation and specialization of individual devices that are part of an intelligent network, as well as techniques for warm-starting intelligent models on new devices in the network, as they come online.
Embedded processors come in all shapes and sizes. Therefore, we are building a compiler that deploys intelligent pipelines on heterogeneous hardware platforms.
Another aspect of our work has to do with making our algorithms accessible to non-experts. Most of the intelligent devices of the future will be invented by innovators who don’t have formal training in machine learning or statistical signal processing. These innovators are part of an exponentially growing group of entrepreneurs, tech enthusiasts, hobbyists, tinkerers, and makers. Developing world-best edge intelligence algorithms is only half the battle—we are also working to make these algorithms accessible and usable by their intended target audience.
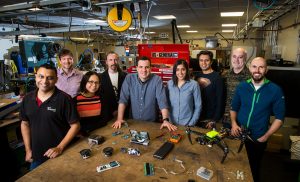
The researchers, in Microsoft’s Redmond, Washington lab, working on the project include, from left to right, Ajay Manchepalli, Rob DeLine, Lisa Ong, Chuck Jacobs, Ofer Dekel, Saleema Amershi, Shuayb Zarar, Chris Lovett and Byron Changuion. Photo by Dan DeLong

The researchers, in Microsoft’s India lab, working on the project include, clockwise from left front, Manik Varma, Praneeth Netrapalli, Chirag Gupta, Prateek Jain, Yeshwanth Cherapanamjeri, Rahul Sharma, Nagarajan Natarajan and Vivek Gupta. Photo by Mahesh Bhat