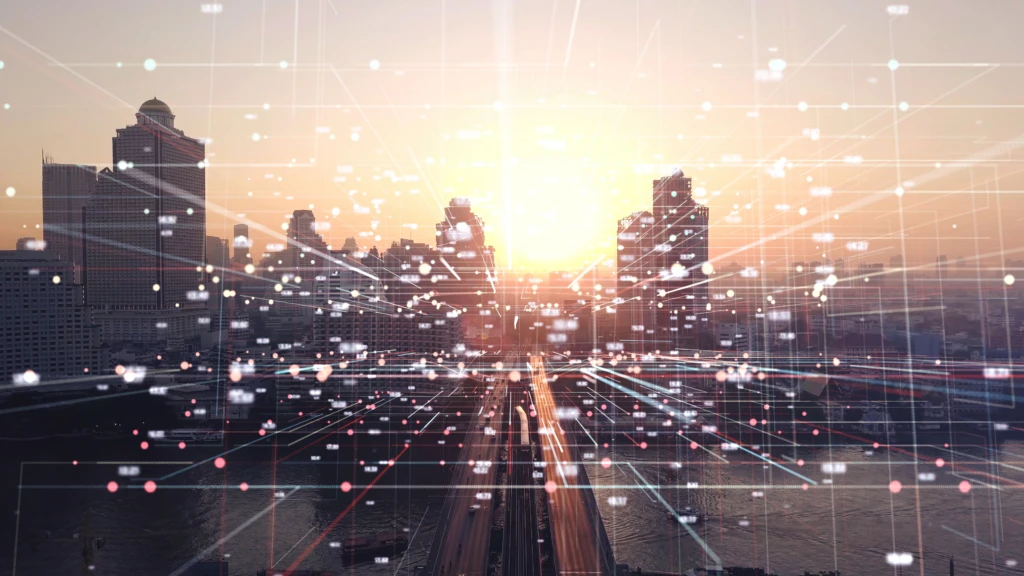
Analytics for fraud prevention and fraud detection in taxes
For more than a decade, tax administrations across the globe have been exploring the use of artificial Intelligence (AI) and machine learning (ML) to prevent and detect tax evasion.
While there are promising results, AI needs to further evolve and mature to drive increased impact. Democratizing access to AI, training more experts in data science and data engineering, and deploying more agile development life cycles are all key for that evolution and progress.
Tax administrations have traditionally used risk-based models applied in batch at the end of the fiscal year to look for discrepancies. AI and machine learning present an exciting opportunity to move to near-real-time detection and reporting of discrepancies on tax data to the government. We invite you to read Microsoft Fraud Protection Capabilities to see how Adaptive AI continuously learns to protect you against payment fraud, bots, account takeover, and returns and discounts fraud. This article introduces account protection and how AI addresses common challenges such as fake accounts, fraudulent account access, and user account abuse.
Changing payment methods
New billing and payment methods are changing the rules in the public finance industry. Billentis¹, recently forecasted that the global market for electronic invoicing is expected to be 550 billion invoices annually and will quadruple in size by 2035. Governments worldwide are pushing e-Invoicing as a mechanism to perform fiscal reporting, with better collection of value-added taxes (VAT) being one of the key drivers.
Existing risk-management and business rule-matching techniques used by tax agencies to run background checks, qualify, and detect fraud and other such tasks can be enhanced by using AI/ML to:
- respond proactively and rapidly to a detected event with near-real-time data.
- integrate more sources of data and share data across the tax ecosystem
- better understand taxpayer reporting and compliance behavior.
- improve the quality of data.
- drive more proactive digital audits and less reactive audits.
How a tax agency can improve its effectiveness in the detection and prevention of fraud
We consider four dimensions to build an organizational capability: people, process, technology, and data.
People—culture and digital skills
Building AI/ML and data science capabilities involve developing qualified human talent with appropriate skills and introduce new ways of getting things done. A culture of innovation and digital skills are fundamental for this change. You can read about what Microsoft offers in digital skills-building in this article: “Microsoft launches initiative to help 25 million people worldwide acquire the digital skills needed in a COVID-19 economy.”
Subject matter experts in the business are key to leveraging the institutional knowledge related to risk management, tax auditing, and tax intelligence. They play a crucial role in the exploratory phases and even more so in interpreting and validating results produced by AI/ML. Business subject matter experts are the ones who finally validate and qualify an AI/ML result as fraud—or not.
Process
For fraud detection and prevention, the process for interpreting results is critical. Once diagnosed, identified anomalies, abnormal behaviors, or potential fraudulent taxable transactions need confirmation through automated techniques with a ‘human in the loop’ validation procedure. Human intervention allows the tax authorities to leverage the organizational knowledge from their in-house subject matter experts and make appropriate interpretations surfaced by the models.
Further, adopting low code application development and process automation can help generate actionable data insights, connect to auditing systems, and automate alerts and notifications. The benefits extend to the productivity of the human talent that participates in the fraud detection process. Here is an inspirational success story of how a “Progressive retailer resolves challenges with low-code automation and AI solutions.”
Technology
An effective practice to detect and prevent fraud demands the use of multiple analytics and machine learning techniques. These include text clustering, entity resolution, risk scoring, network analysis, and others. They are selectively used to do taxpayer profiling and segmentation, risk scoring of tax operations, analysis of the supply chain, and identification of abnormal transactions among many others.
Security and privacy are genuine concerns for government agencies when storing data in the public cloud. The evolution of confidential computing and other technologies are enabling strong isolation of sensitive data while at rest and during processing in the cloud.
Investing in agile development practices such as , MLOps, and DataOps are essential for the efficient deployment, testing, calibration, and operationalization of machine learning algorithms. This is foundational for introducing effective fraud detection on tax agency data. You can read the “GigaOm-Delivering on the Vision of MLOps” report to familiarize yourself with the MLOps practice.
As tax agencies collect more types and larger amounts of data such as e-Invoicing, capabilities in the hyper-scale cloud provide a backbone to deal with the volume, variety, and velocity of such data. Big Data solutions in the cloud provide the flexibility, performance, and efficiency required to do near-real-time data processing.
The following figure illustrates the challenge of increasing volumes of data when e-Invoicing is introduced.
Data
And finally, the importance of good data that is critical to achieving the best results. That said, developing good-quality data is not simple. However, the introduction of near-real-time analytics helps detect quality gaps and introduce preventive practices, such as alerting the taxpayer for inconsistencies. Conventional analytics processes run after the fact, months or maybe even years later, limiting the opportunity to fully exploit data for its potential. That is why near-real-time analytics is highly valuable for Tax Agencies.
A major opportunity is the ability to drive value from data as fast as possible. Near-real time analytics should be part of the AI agenda. According to the Organisation for Economic Co-operation and Development (OECD): ‘The advent of a digital age in government has created new opportunities for assessing risks of fraud and corruption in infrastructure.’ ²
AI maturity
As we mentioned at the beginning of this article, the adoption of artificial intelligence and machine learning is a journey, moving through multiple levels of maturity. As you can see in the figure below, Microsoft has identified four maturity levels for tax administrators to adopt AI and ML.
A comprehensive AI platform is a key component for fraud detection and fraud prevention in the tax administration.
Summary
Speedy responses are critical for detecting and preventing fraud. Newer and fast emerging tax-evasion schemes introduce higher levels of sophistication and complexity that departments need to address constantly. The digitalization of taxable transactions has become a reality. All this presents a very real opportunity for tax administrators to increase the use of artificial intelligence to use data proactively and at near-real-time to fight against fraud.
A comprehensive approach for enhancing AI, acquiring human talent, creating AI processes, implementing an AI platform, and improving the use of tax data, is therefore fundamental.
We invite you to read more about the Microsoft Fraud Protection capabilities to see Adaptive AI that continuously learns to protect you against payment fraud, bots, account takeovers, and returns and discounts fraud. This provides a good source of inspiration to introduce fraud prevention and protection in Tax .
To learn more about Microsoft’s global view in Government, visit our web page
¹ Bellentis, The e-invoicing journey 2019-2025, Fourth Edition, September 2019
² Analytics for Integrity: Data driven Approaches for Enhancing Corruption and Fraud Risk Assessments, OECD 2019