Disaster response and building for resiliency
Every year natural disasters like flooding, hurricanes, tornadoes, and fire put millions across the globe at risk and cause losses worth trillions.1 Climate change is expected to drive up the pace and severity of these events. The AI for Good Lab is currently doing a lot of work to combine geospatial data, like high-resolution satellite imagery, with innovative machine learning techniques to analyze the impacts of disasters, which can help governments respond once an event has happened. But we are going beyond that, developing tools to help predict natural disaster impacts ahead of time, making it possible to better target efforts to prevent loss of life and mitigate damage.
Predicting cyclone damage and heat wave impacts in India
One example is our work with SEEDS (opens in new tab), a nonprofit disaster response and preparedness in India, which received a grant in 2019 from Microsoft for financial and technical support to mitigate damage from cyclones. The path of a devastating cyclone can be predicted 48 hours or so of its arrival, but manually collecting information about houses in its path is very time intensive. The Lab helped SEEDS develop an AI model, called “Sunny Lives”, using high-resolution satellite imagery of the areas likely to fall in the cyclone’s path and applied advanced data analytics and machine learning to identify the houses at highest risk. The model was piloted for risk assessment of cyclone induced flooding during cyclone Nivar and cyclone Burevi in 2020 and showcased promising results. The information provided by the model and risk scoring pipeline enabled SEEDS and its on-ground partners to target outreach to those communities with measures such as distributing an advisory in multiple languages to thousands the day before a cyclone arrived.
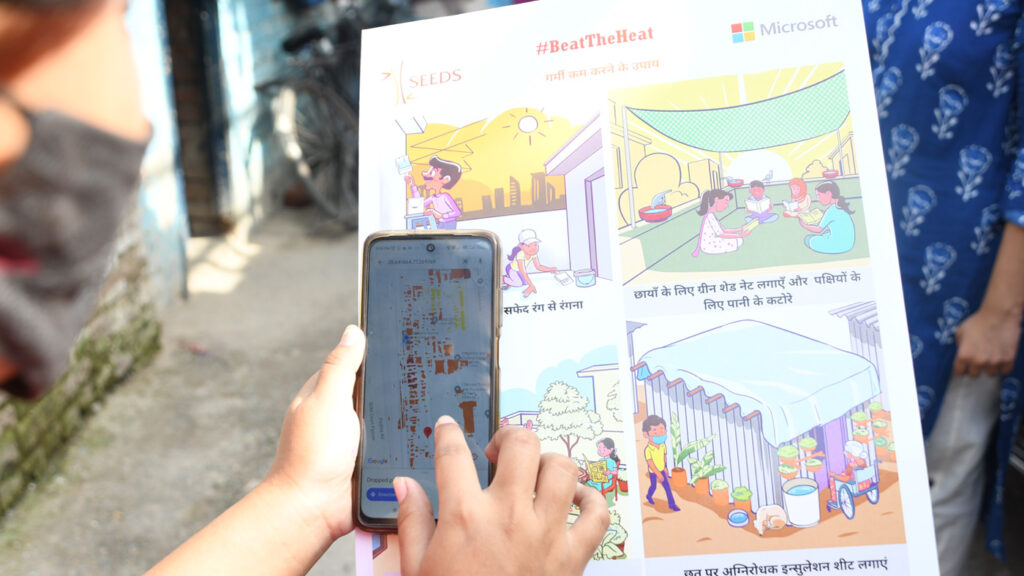
In another project in 2022, the Lab worked with SEEDS to refine the AI model to generate risk information for heat waves in India. India has seen twice as many heat waves between 2000-2019 as between 1980-1999.2 The AI model focused on heat waves affecting around 125,000 people living in slums in New Delhi and Nagpur. The Lab leveraged data from local risk maps developed by a partner that scored buildings with such parameters as built-up density, vegetation, proximity to a water body and rooftop material. The risk map is overlaid onto a regular map which can then be displayed on a smartphone, helping teams in the field figure out where to issue warnings, or where the local authorities need to direct resources. Microsoft President Brad Smith noted at the Web Summit (opens in new tab) that the work done in collaboration with SEEDS is “an extraordinary combination of high-tech and low-tech coming together… It’s part of what the world needs to do to adapt to climate change.”
Expediting damage assessments for the Red Cross
The Lab is currently working together with the American Red Cross on disaster response by applying our current Microsoft damage assessment tool to the Red Cross’s data on hurricanes and tornadoes in the U.S. from 2022. The research aims to investigate preliminary impact and damage assumptions to determine ways to improve and expedite insights that can help inform ground operations.
Addressing food insecurity with machine learning

Millions of people worldwide face chronic food insecurity. Nearly 828 million people were hungry in 2021, or 9.8% of the world population, according to the Food and Agriculture Organization of the United Nations1. In order for aid workers and governments to prepare for and deliver timely assistance, early warning systems are needed to help them quickly forecast the incidence of hunger as conditions change due to natural disasters, war and other factors.
In 2021, the Lab conducted a study jointly with the Catholic Relief Services (CRS) to apply machine learning techniques to this problem. Data from household surveys performed on the ground in southern Malawi by CRS teams was combined with machine learning algorithms, creating a model to predict future food insecurity at the household level in near real-time. The model proved to have an 83% accuracy rate in predicting food security outcomes and could generate accurate forecasts up to 4 months in the future.
Using AI to address inequities in public health
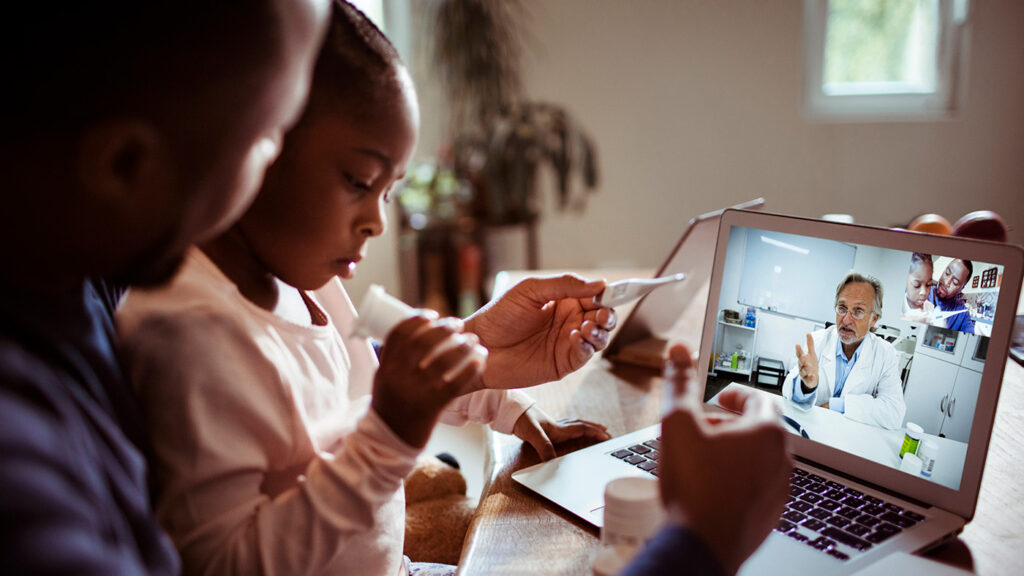
A huge need exists to address inequities in health and healthcare for underserved populations, and to reduce disparities between populations across the globe. Microsoft is committed to combatting health disparities based on gender, race, ethnicity, or income level. In 2020, Microsoft invested $60 million in AI for Health, a five-year project to apply AI to improving global health. To read about the Lab’s role in this work, visit AI for Health | Microsoft AI.
The Lab’s current work looks deeply at inequities in public health risks and disease prevalence in rural areas. Disparities in healthcare access, health status, screening, and broadband access lead to less healthy outcomes for rural dwellers compared to urban. A great deal of data is available about health risk behaviors, disease prevalence, health status, health services utilization, healthcare quality, local economic conditions and health outcomes, but no easy way to compare local data between non-metro and metro areas.
The Lab is developing a visualization tool, known as the Rural Health Index Map, to assist policymakers in understanding health disparities at the county and zip code levels. Embedded analytics will allow for simulations, showing how changing a parameter, such as a risk factor or economic distress, might impact health outcomes. This tool might help policymakers in a number of ways, by providing insights that help them decide on investments to improve local health status, for example, or to see how changes to rural broadband access can potentially impact public health.
Footnotes
1 Disaster Relief and Rehabilitation (SEEDS) (opens in new tab)