Intelligent machines and intelligent software rely on algorithms that can reason about observed data to make predictions or decisions that are useful. Such systems rely on machine learning and artificial intelligence, combining computation, data, models, and algorithms. Our mission, in the Machine Intelligence theme at Microsoft Research Cambridge, is to expand the reach and efficiency of machine intelligence technology.
We research how to incorporate structured input data such as code and molecules effectively into deep learning models. We invent new methods so models can accurately quantify their uncertainty when making predictions. We build models that learn from small data that is corrupted or only partially observed. We develop deep learning algorithms that apply to interactive settings in gaming and in decision making task, where model predictions have consequences on future inputs.
Improving the performance of machine learning methods demands an ever-increasing scale in computation while retaining flexibility to develop new models. We research new AI compiler technology that will make it easier to express rich algorithms while effectively utilizing modern accelerators.
Projects
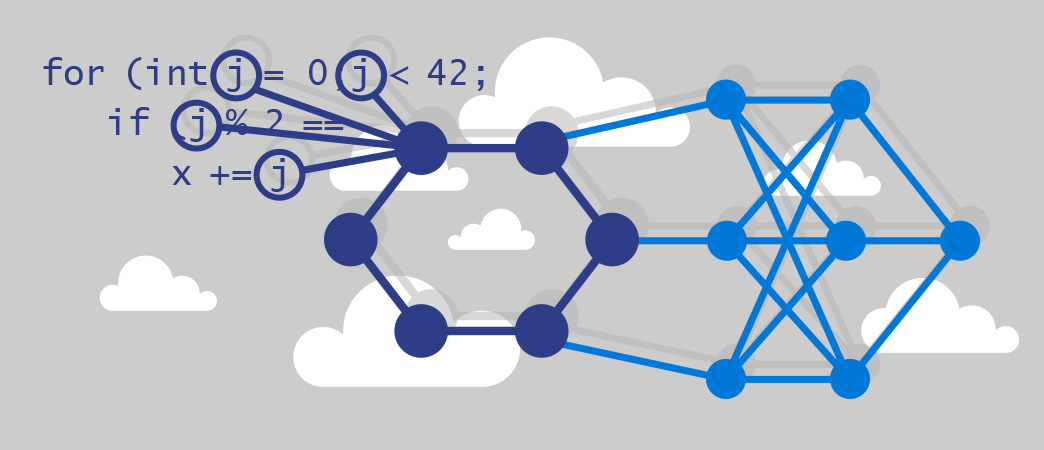
Deep Program Understanding
This project aims to teach machines to understand complex algorithms, combining methods from the programming languages, software engineering and the machine learning communities.
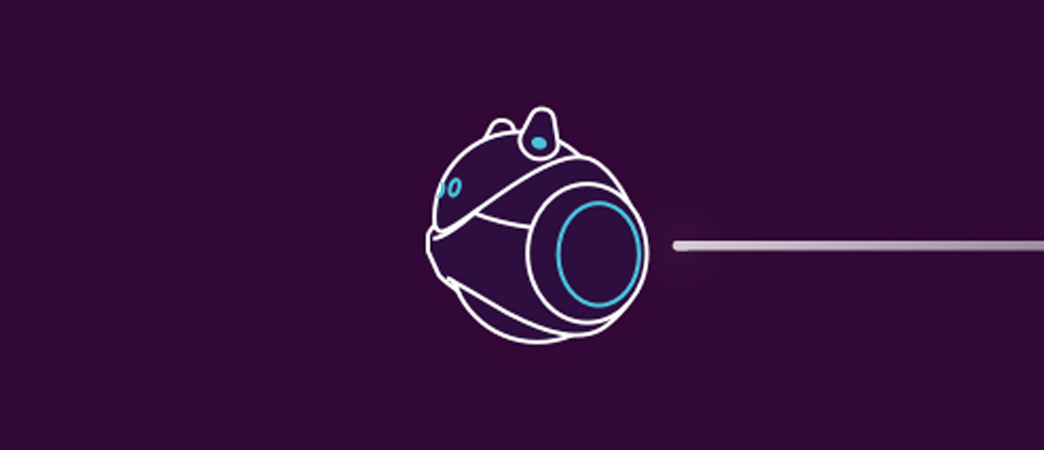
Deep Reinforcement Learning for Games
We aim to teach machines to understand complex algorithms, combining methods from the programming languages, software engineering and the machine learning communities.
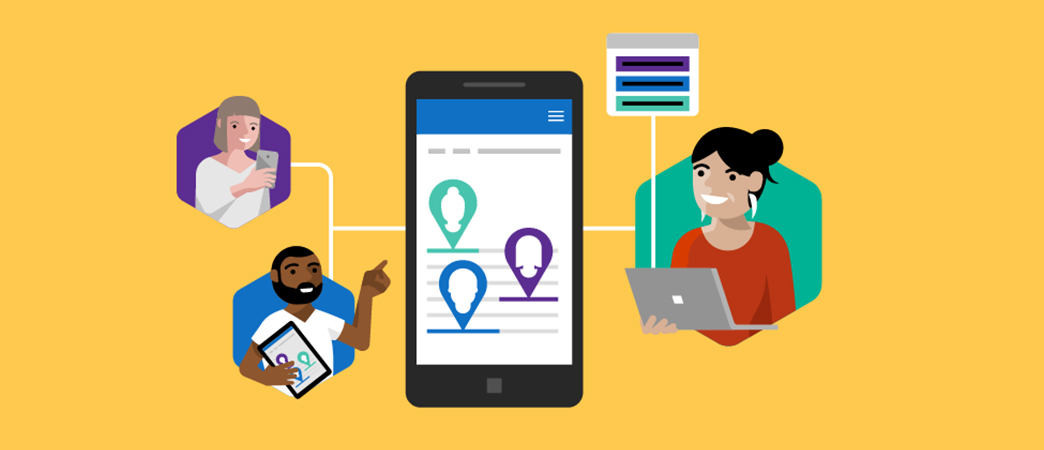
Enterprise Knowledge
The aim of the Enterprise Knowledge project is to automatically extract business knowledge into a single, consistent knowledge base, made up of the entities that really matter to each organisation.

Infer.Net
Infer.NET is a .NET library for machine learning. It provides state-of-the-art algorithms for probabilistic inference from data. Infer.NET is open source software under the MIT license.
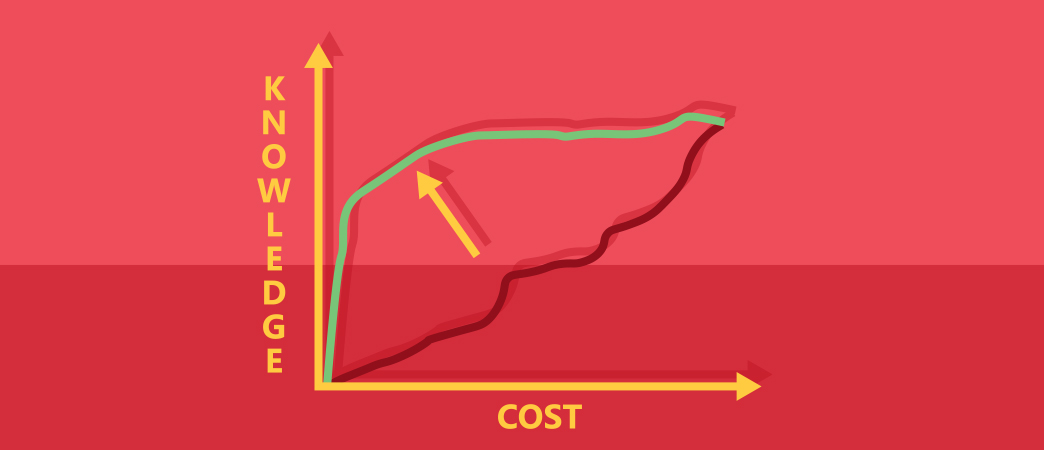
Project Causica
In this project we investigate how to best utilize AI algorithms to aid decision making while simultaneously minimizing data requirements (and, therefore, cost).
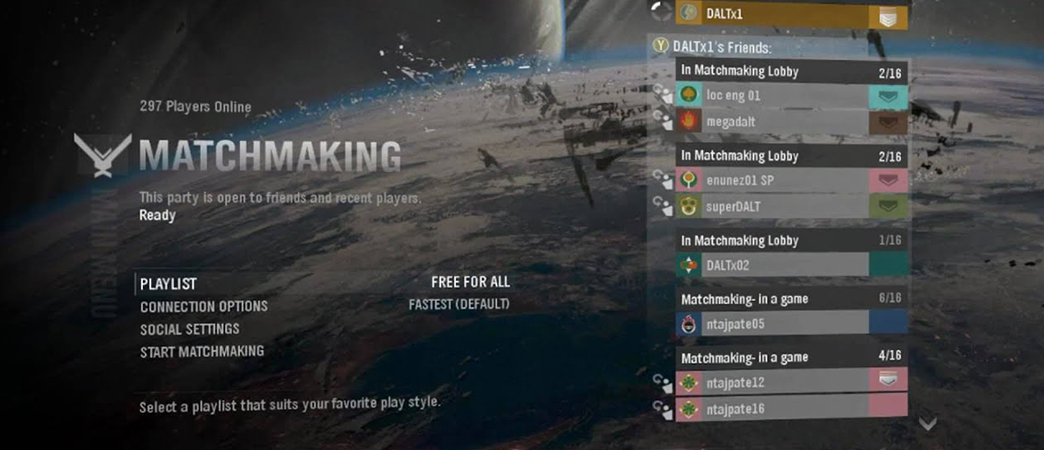
TrueMatch
The TrueMatch matchmaking system decides which people should play together in an online multiplayer game. The Coalition have announced (opens in new tab) that Gears 5 will use TrueMatch.
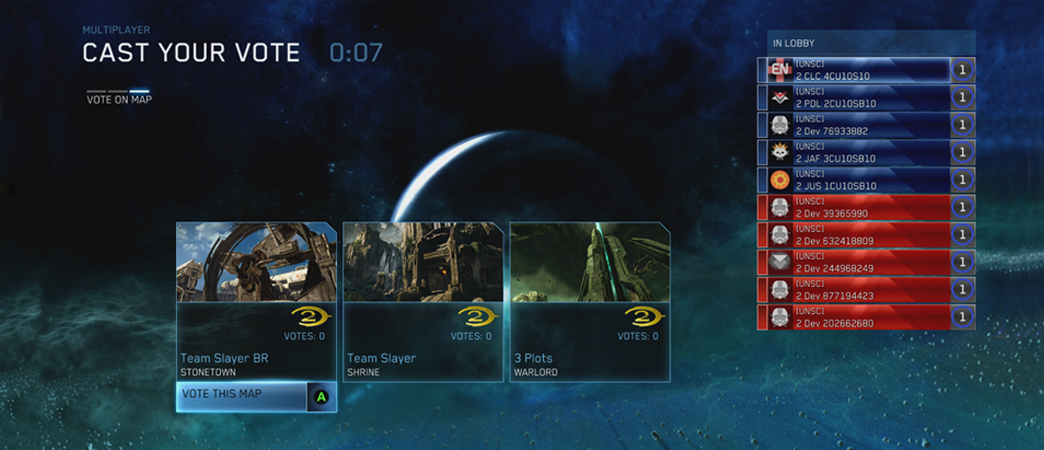
TrueSkill
The TrueSkill ranking system is a skill-based ranking system designed to overcome the limitations of existing ranking systems, and to ensure that interesting matches can be reliably arranged within a league.
People
Dave Bignell
SR RESEARCH SCIENTIST
Sam Devlin
Principal Researcher
Adam Foster
Raluca Georgescu
Senior Research Scientist
Wenbo Gong
Senior Researcher
Tarun Gupta
AI Researcher
Katja Hofmann
Senior Principal Researcher
Sarah Lewis
Senior Research Engineer
Chao Ma
Krzysztof Maziarz
Senior Applied Researcher
Tom Minka
Senior Principal Researcher
Pavel Myshkov
Senior Researcher
Hannes Schulz
Senior Researcher
Marwin Segler
Principal Researcher
Shanzheng Tan
Researcher / Technical Program Manager
Jonathan Tims
Senior Software Development Engineer
Ryota Tomioka
Principal Research Manager
Sam Webster
Senior Software Development Engineer
Tian Xie
Principal Research Manager
Yordan Zaykov
Principal Research Engineering Manager
Rianne van den Berg
Principal Research Manager